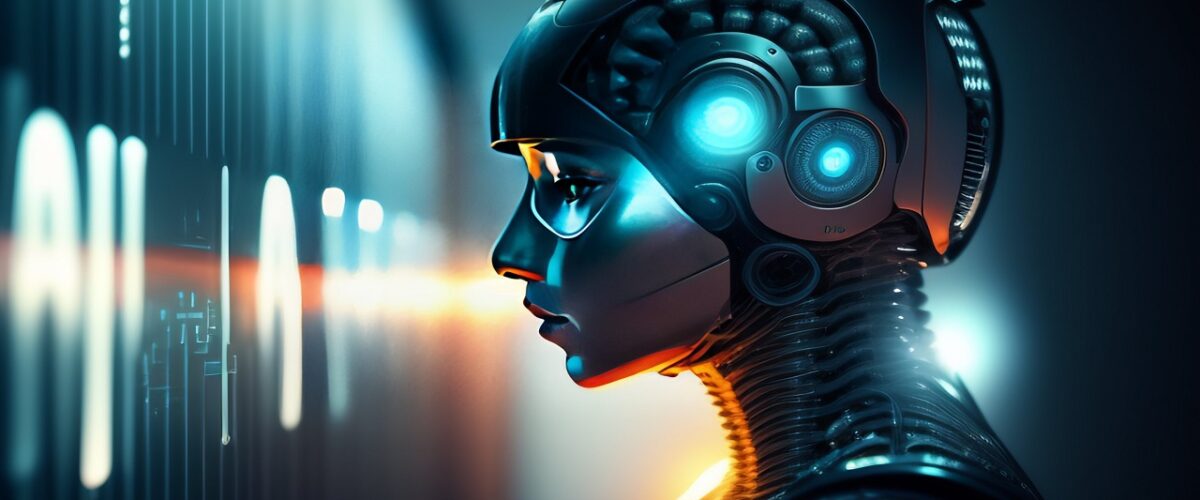
Very rarely do technological releases “break the internet” in the way that popular culture can (what colour was that dress again? ), but the release of ChatGPT just 4 months ago has pushed itself so deeply into the collective consciousness that it already feels like “generative” and “AI” are two words we’ve all been using in close proximity to one another since time immemorial!
By any metric, the team at OpenAI’s achievements, both in the Large Language Model (LLM)-based technology itself and in how efficiently they were able to promote virtually instantaneous worldwide acceptance, are genuinely extraordinary. It does an amazing job of producing extremely fluent, mostly correct, and highly useful answers to any question you ask it on essentially any topic, with a user interface that is super simple and accessible, resulting in the mainstreaming of language model AI technology. Everyone is now attempting to figure out how to use this new set of “superpowers” to increase production and efficiency in all sectors and areas of life.
However, language models are nothing new in the realm of Natural Language Processing. According to a report released in 2017 by a group of Googlers (Vaswani et al), the main breakthrough that lead to ChatGPT is the “transformer” model architecture, which employs a deep neural network with encoder/decoder architecture and self-attention mechanisms. Around the same time as that paper, this same innovation drove the transition from statistical machine translation to neural machine translation (NMT), with Google Translate beginning its transition to NMT in 2016, delivering a step change in machine translation quality that remains the foundational technology for state-of-the-art machine translation today.
ChatGPT can also translate, although it is not as powerful or as effective. NMTs and LLMs are not the way of the future for website translation. While both NMTs and LLMs are transformer-based models trained to generate a response to a prompt, the nature of the prompts and responses they are trained to handle, as well as the volume of training data used, and the cost of training, all of which are many orders of magnitude higher for LLMs than for NMTs. LLMs are really valuable multi-purpose tools, much like Swiss Army knives and duct tape, but if you want to stick a nail in the wall, you need a hammer.
When compared to LLMs, NMTs provide significantly superior economics for providing the greatest possible quality of translation across high, medium, and low resource languages, particularly when including unique brand voice requirements such as adherence to style standards and glossaries.
The Swiss Army knife can be used to build a better hammer while also doing some things that hammers can’t (e.g. transcreation-writing new content with the same objective as some existing content within a different cultural context and language).
Building Better Hammers
The process of building a high quality NMT engine has two main stages:
- Establish a Base “Generic” model for the language pair. Either building and training from scratch or using a pre-trained model.
- Domain-adaptation. Fine-tuning the “Generic” model with more specific training data to make it perform better within a given domain (such as adopting the vernacular of an industry segment of the brand voice of a specific company).
While LLMs such as ChatGPT can perform translation at a quality level comparable to generic NMT models (e.g., Google Translate), for most organisations, the more relevant comparison is to a well-trained domain-adapted NMT model, which will produce much better translation quality, much faster, and much cheaper. This is due to the fact that the model size (number of parameters in the neural net) and volume of training data that must be prepped and then used are so much larger in an LLM that the computational cost of both ongoing training and inference (doing a translation) are orders of magnitude higher than in NMTs. This is also why they are significantly slower than NMTs.
LLM models are useful for data augmentation, which is the generation of synthetic training data to supplement real training data chosen for the purpose of training NMTs. This is especially beneficial in medium to low resource languages when finding enough aligned sentence pairs to train an efficient NMT is difficult. The LLM may have enough understanding of the target language to generate synthetic data to supplement your real data, so that an NMT trained with the enhanced data outperforms one trained with only your real data.
Similarly, it may be used to produce synthetic data for domain-adaptation training when there is insufficient real data to perform successful domain-adaptation training.
Our NMT team at MotionPoint has been training brand-adapted NMT models for our customers for some time now, and we are seeing very significant increases in the quality of output from our brand-adapted models when compared to the generic models that we started with, as we use a variety of techniques to source, enhance, and clean training data sets.
Post a Comment