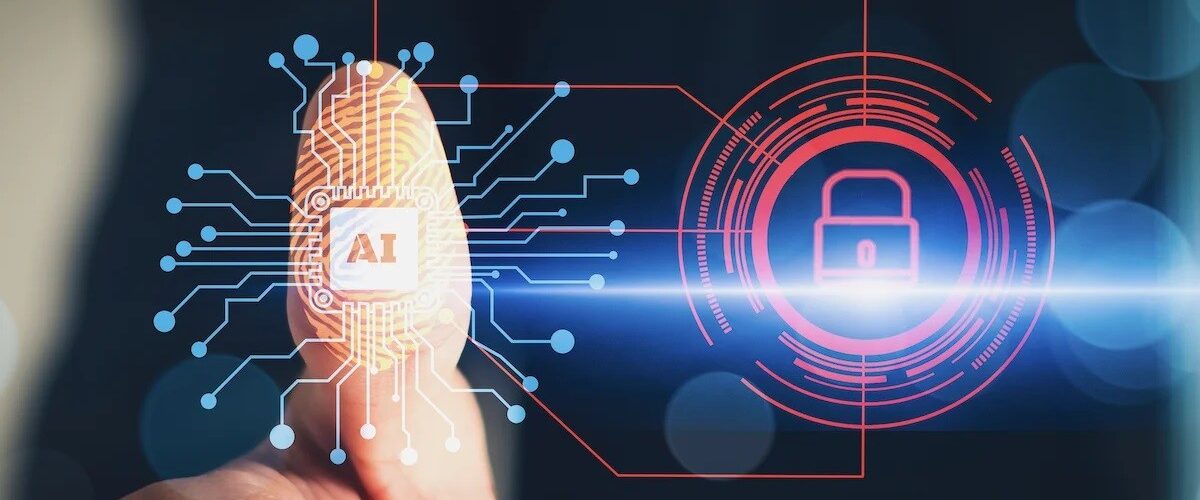
Data privacy in generative AI is an essential consideration when developing and deploying AI models that generate content, such as images, text, or videos. As generative AI models become more advanced and capable of generating highly realistic and personalized content, it is crucial to address the privacy implications and protect sensitive information.
Data privacy in generative AI refers to the protection of personal or sensitive data used to train or generate content with AI models. It encompasses safeguarding individuals’ identities, preserving confidentiality, and preventing unauthorized access, use, or disclosure of personal information.
Generative AI models often require large datasets to learn and generate content effectively. These datasets can contain various types of sensitive information, such as personal images, text, or demographic details. Therefore, it is essential to implement robust privacy measures to mitigate the risks associated with the use and storage of this data.
Protecting Data Privacy in Generative AI Involves Several Key Considerations
- Data Anonymization: Anonymizing the data used for training generative AI models is crucial to protect individuals’ identities. This involves removing or obfuscating personally identifiable information (PII) from the dataset, such as names, addresses, or social security numbers, to ensure that the generated content cannot be linked back to specific individuals.
- Secure Data Storage and Transmission: Implementing secure data storage and transmission protocols is vital to prevent unauthorized access or data breaches. Encryption techniques, access controls, and secure storage systems can help protect the privacy of the training data and generated content.
- Consent and Transparency: Obtaining informed consent from individuals whose data is used in generative AI training is essential. Users should be made aware of how their data will be used and have the option to opt out if they wish. Transparent communication about data usage and privacy practices is crucial in building trust and ensuring individuals’ privacy rights.
- Minimization of Data Collection: Limiting the collection of unnecessary or excessive data can help reduce privacy risks. By collecting only the minimum required data for training generative AI models, the potential impact of a data breach or misuse can be minimized.
- Ethical Use of Data: Adhering to ethical guidelines and regulations, such as data protection laws, is essential when working with generative AI models. Respecting individuals’ privacy rights and ensuring the lawful and responsible use of data are paramount to maintaining public trust.
- Accountability and Transparency: Implementing mechanisms for accountability and transparency in the development and deployment of generative AI models can help ensure compliance with privacy standards. Regular audits, documentation of data handling processes, and clear policies on data privacy contribute to maintaining ethical practices.
Data privacy in generative AI is an ongoing and evolving concern, especially as AI models become more sophisticated and capable of generating increasingly realistic content. It requires a holistic approach that combines technological measures, legal compliance, and ethical considerations to protect individuals’ privacy while fostering innovation and advancement in generative AI.
User Consent of Data Privacy in Generative AI
User consent plays a crucial role in ensuring data privacy in generative AI. It involves obtaining explicit permission from individuals whose data is used in training generative AI models or generating content. User consent serves as a legal and ethical foundation for the responsible collection, use, and storage of personal or sensitive data.
When it comes to generative AI, user consent should cover the following aspects:
- Data Collection: Clearly inform users about the types of data that will be collected, including the specific data points or attributes required for training the generative AI model. This may include images, text, audio, or other relevant data. Users should be aware of the purpose and scope of data collection.
- Data Usage: Provide a clear explanation of how the collected data will be used. Inform users that their data will be used to train generative AI models and generate content. Transparency is crucial in ensuring that users understand how their data contributes to the development and functionality of the AI system.
- Data Storage and Retention: Inform users about the duration for which their data will be stored and retained. Specify the measures in place to protect the data from unauthorized access or breaches. Users should be aware of the security measures employed to safeguard their data during storage.
- Data Sharing: If there is any intention to share user data with third parties, it is important to obtain explicit consent for such sharing. Clearly state the purpose and recipients of the shared data. Users should have the option to opt-in or opt-out of data sharing, depending on their comfort level and preferences.
- Withdrawal of Consent: Allow users the right to withdraw their consent at any time. Inform them of the process to revoke their consent and the implications of doing so. Users should have control over their data and be able to exercise their rights regarding its usage.
- Communication and Updates: Maintain open lines of communication with users regarding any changes to the data privacy policy or terms of use. Notify users about updates, modifications, or additions to the generative AI system’s functionality that may impact their data privacy.
Obtaining explicit and informed consent is crucial to respect users’ privacy rights and ensure compliance with data protection regulations. It establishes a transparent relationship between the generative AI system and the users, fostering trust and accountability.
It is important to note that obtaining user consent is just one aspect of data privacy. Implementing robust security measures, anonymizing data, and adhering to legal and ethical standards are equally important to protect user data and ensure responsible use of generative AI models.
Data Ownership of Data Privacy in Generative AI
Data ownership in generative AI is a complex and evolving topic that requires careful consideration. It involves determining who has the rights and control over the data used to train generative AI models and generate content. Data ownership in generative AI can have implications for data privacy, intellectual property, and ethical considerations.
In the context of generative AI, data ownership can be approached from different perspectives:
- User Data Ownership: User data used to train generative AI models, such as images, text, or personal information, typically belongs to the individuals who provide it. Users should have control over their own data and the right to determine how it is used. Respect for user data ownership entails obtaining explicit consent, providing transparency about data usage, and allowing users the ability to manage and revoke their consent.
- Data Creator Ownership: In cases where individuals generate or create data using a generative AI system, such as artwork or text, the ownership of that generated content can be a nuanced topic. The ownership rights may depend on applicable copyright laws and the terms of use or licensing agreements associated with the generative AI system. Clear guidelines and agreements can help establish the ownership and usage rights for the generated content.
- Data Aggregator or Platform Ownership: In some instances, data used to train generative AI models may be collected and owned by data aggregators or platforms. These entities may have the right to control, store, and utilize the data within the bounds of applicable laws and user agreements. Data aggregators and platforms should adhere to data privacy regulations, respect user consent, and provide transparent policies regarding data ownership and usage.
- Shared Ownership or Collaboration: In certain scenarios, data ownership can be shared among multiple parties involved in collaborative projects or research endeavors. Ownership rights can be determined through agreements or contracts that outline the responsibilities, usage rights, and data sharing arrangements among the involved parties.
Determining data ownership in generative AI is not always straightforward and can depend on various factors, including legal frameworks, user agreements, and the specific context of data collection and usage. It is important to establish clear guidelines, ethical considerations, and legal frameworks to navigate data ownership and ensure fair and responsible practices in the development and deployment of generative AI models.
Additionally, it is essential to respect data privacy rights, protect sensitive information, and address ethical concerns, regardless of data ownership. Striking a balance between data ownership, privacy, and ethical considerations is crucial to foster trust, promote responsible data practices, and ensure the fair and ethical use of generative AI technologies.
Conclusion
In conclusion, data privacy is of paramount importance in the field of generative AI. As generative AI models become more advanced and capable of generating highly realistic and personalized content, protecting sensitive data and preserving individuals’ privacy rights become crucial considerations.
Data privacy in generative AI ensures that personal or sensitive information used for training models or generating content is handled responsibly, with appropriate safeguards in place. It involves obtaining explicit user consent, anonymizing data, implementing secure storage and transmission protocols, and adhering to legal and ethical guidelines.
Post a Comment