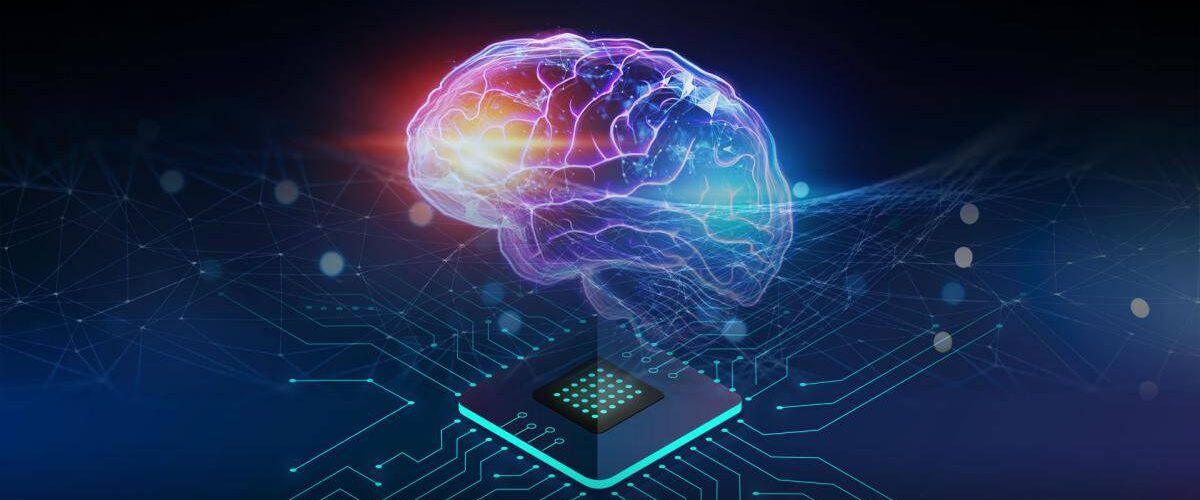
Generative AI techniques have revolutionized the field of image processing and manipulation, particularly in the areas of image-to-image translation and style transfer. These techniques enable the transformation of images from one domain to another, or the application of artistic styles to images, all achieved through advanced generative models.
Image-to-image translation involves transforming an input image from one domain to another while preserving its content. For example, it can convert a sketch into a realistic image, transform a day scene into a night scene, or turn a horse into a zebra. Generative adversarial networks (GANs) and conditional GANs (cGANs) are commonly used to accomplish these tasks. By training on paired examples from both domains, these models learn to capture the underlying structure and mapping between the source and target domains, enabling them to generate realistic and visually coherent translated images.
Style transfer, on the other hand, focuses on applying the artistic style of one image to another while preserving the content. It allows users to transform an image into various artistic styles, such as paintings by famous artists like Van Gogh or Picasso. Style transfer models leverage deep neural networks, typically based on convolutional neural networks (CNNs), to separate and recombine the content and style of images. By extracting high-level features from both the content image and the style reference image, these models can generate a new image that preserves the content of the input image while incorporating the desired artistic style.
Generative AI techniques for image-to-image translation and style transfer have numerous practical applications and creative possibilities. They can be used in areas such as augmented reality, virtual reality, computer graphics, and creative design. Image-to-image translation can aid in domain adaptation, image synthesis, and data augmentation for machine learning tasks. Style transfer provides a means for artists, designers, and photographers to explore new creative expressions and generate visually captivating artwork.
In this rapidly evolving field, researchers and developers are continuously exploring new architectures, loss functions, and training strategies to improve the quality and versatility of generative models for image-to-image translation and style transfer. These advancements are driving the boundaries of what is possible in image manipulation and opening up exciting opportunities for both practical applications and artistic endeavors.
Benefits of Using Image-to-Image Translation and Style Transfer
Using image-to-image translation and style transfer techniques offers several benefits, making them valuable tools in various domains. Here are some of the key advantages:
- Creative Expression and Artistic Exploration: Image-to-image translation and style transfer enable artists, designers, and photographers to experiment with different visual styles and create unique and captivating artwork. These techniques provide a means to apply the characteristics of renowned artists or artistic movements to their own images, allowing for novel and personalized expressions of creativity.
- Rapid Prototyping and Design Iteration: Image-to-image translation facilitates the quick generation of variations of an image in different domains. This is particularly useful in design fields, allowing designers to visualize different possibilities and iterate rapidly. For example, in architecture, it can help visualize buildings in different styles or materials, aiding in the decision-making process.
- Domain Adaptation and Data Augmentation: Image-to-image translation techniques can be employed for domain adaptation tasks, where the source and target domains share similar underlying structures but have different appearances. By translating images from the source domain to the target domain, it becomes possible to train machine learning models using augmented datasets, improving their performance in the target domain.
- Data Generation and Synthesis: Image-to-image translation models can generate new images that adhere to specific domain constraints. For instance, these models can synthesize images of virtual characters, objects, or scenes that align with desired characteristics. Such synthesized data can be valuable for various applications, including gaming, virtual reality, and computer graphics.
- Aesthetic Enhancement and Visual Transformations: Style transfer techniques allow for the application of artistic styles to images, enhancing their visual appeal and evoking different emotions. This can be beneficial in fields such as advertising, marketing, and visual media, where the aesthetics and impact of images play a significant role in engaging audiences.
- Augmented Reality and Virtual Reality Experiences: Image-to-image translation and style transfer can enrich augmented reality (AR) and virtual reality (VR) experiences by dynamically transforming the visual appearance of real-world scenes or virtual objects in real-time. This enables users to immerse themselves in unique environments or interact with digital content in various visually compelling ways.
- Data Compression and Bandwidth Optimization: By leveraging image-to-image translation, it is possible to represent and transmit images more efficiently. By translating images into a compressed domain or a simplified representation, the size and bandwidth requirements can be reduced, making it easier to transmit and store images with minimal loss in visual quality.
- Preservation of Privacy and Confidentiality: Style transfer can be used to transform images while preserving privacy-sensitive content. For instance, anonymizing faces or personal information in images through style transfer techniques allows for the sharing or publication of images while protecting individuals’ identities.
These benefits highlight the versatility and practical applications of image-to-image translation and style transfer techniques. As research and development in this field continue to progress, we can expect further advancements and wider adoption across industries, enabling new possibilities for creative expression, data manipulation, and visual enhancement.
Conclusion
In conclusion, generative AI techniques for image-to-image translation and style transfer have revolutionized the field of image processing, offering numerous benefits and creative possibilities. These techniques enable the transformation of images from one domain to another, or the application of artistic styles to images, through advanced generative models.
The advantages of using image-to-image translation and style transfer techniques are multifaceted. They empower artists, designers, and photographers to explore new creative expressions and generate visually captivating artwork. Rapid prototyping and design iteration become possible, allowing for quick visualization of different possibilities and facilitating decision-making processes.
These techniques also find utility in domain adaptation and data augmentation tasks, where they assist in improving the performance of machine learning models by translating images from a source domain to a target domain. Moreover, image-to-image translation enables data generation and synthesis, providing valuable datasets for applications such as gaming, virtual reality, and computer graphics.
Style transfer techniques enhance the aesthetic appeal of images, enabling the application of artistic styles and evoking different emotions. They contribute to visual transformations, augmenting the impact of images in fields like advertising, marketing, and visual media. Additionally, image-to-image translation and style transfer enhance augmented reality and virtual reality experiences, allowing for dynamic visual transformations in real-time.
Moreover, these techniques have practical implications such as data compression and bandwidth optimization, enabling efficient representation and transmission of images. They also facilitate the preservation of privacy and confidentiality by anonymizing sensitive content while preserving the overall appearance of the image.
As research and development in this field progresses, we can anticipate further advancements and wider adoption of generative AI for image-to-image translation and style transfer. These techniques continue to push the boundaries of creative expression, data manipulation, and visual enhancement, enabling new possibilities in various industries and domains.
Post a Comment