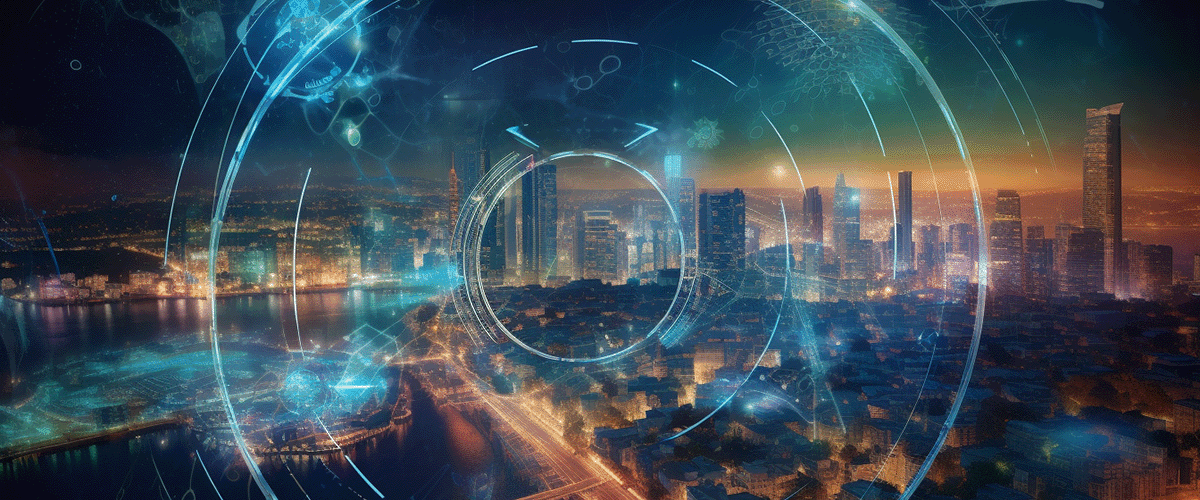
Generative AI systems, which utilize artificial intelligence algorithms to generate new content such as images, text, or music, have gained significant attention for their creative capabilities. However, as these systems become more sophisticated and prevalent, it becomes imperative to evaluate their fairness and interpretability. Fairness ensures that generative AI systems do not perpetuate biases or discriminate against individuals or groups, while interpretability focuses on understanding how the system arrives at its outputs.
Evaluating the fairness of generative AI systems involves examining the potential biases and ethical implications embedded in the training data and algorithms. If the training data is biased or skewed towards certain demographics or contains discriminatory patterns, the generated content may reflect these biases. It is essential to assess and mitigate such biases to ensure fairness and prevent harm to marginalized groups.
Interpretability, on the other hand, addresses the need to understand how generative AI systems make decisions or generate outputs. Interpretability allows users and developers to gain insights into the underlying processes and ensure transparency, accountability, and trust in the system’s operations. It enables users to understand how the system arrived at a particular output and helps identify any potential errors or biases.
Several evaluation approaches can be employed to assess fairness and interpretability in generative AI systems. Fairness assessments may involve analyzing the training data for biases, testing the system’s outputs against different demographic groups, or implementing post-processing techniques to correct biases. Interpretability evaluations may involve techniques such as generating explanations for the system’s outputs or using visualization methods to understand the decision-making process.
Addressing fairness and interpretability challenges requires interdisciplinary collaboration between AI researchers, ethicists, domain experts, and affected communities. It involves developing robust evaluation metrics, creating diverse and representative datasets, and implementing algorithms and techniques that promote fairness and interpretability. Additionally, regulatory frameworks and guidelines can play a vital role in guiding the evaluation and deployment of generative AI systems, ensuring they align with societal values and ethical standards.
In this exploration of evaluating the fairness and interpretability of generative AI systems, we will delve into the challenges, methodologies, and best practices in assessing these critical aspects. We will examine the importance of diverse and representative training data, transparency in algorithmic decision-making, and the role of user feedback and community engagement in fostering fairness and interpretability. By evaluating and improving the fairness and interpretability of generative AI systems, we can promote trust, accountability, and ethical use of these technologies in a wide range of applications, from art and entertainment to healthcare and social decision-making.
Benefits of Fairness and Interpretability of Generative AI Systems
The fairness and interpretability of generative AI systems offer several significant benefits. By ensuring fairness and improving interpretability, we can enhance the trustworthiness, accountability, and ethical use of these systems. Here are some key advantages:
- Mitigating Bias and Discrimination: Fairness evaluation helps identify and mitigate biases in generative AI systems. By addressing biases present in training data or algorithms, we can prevent the generation of content that perpetuates stereotypes or discriminates against certain individuals or groups. This fosters inclusivity and reduces the potential harm caused by biased outputs.
- Enhancing User Trust and Acceptance: Fairness and interpretability are vital for building user trust in generative AI systems. When users understand how the system works and can verify that it operates fairly and without hidden biases, they are more likely to trust and accept the generated outputs. This increased trust facilitates user adoption and engagement with generative AI systems.
- Ensuring Accountability and Responsible AI Use: By making generative AI systems more interpretable, we can hold them accountable for their outputs and decisions. Interpretability allows users, developers, and regulators to understand the factors influencing the system’s outputs, enabling responsible use and ensuring compliance with ethical guidelines and legal frameworks. This promotes transparency and responsible AI development and deployment.
- Facilitating Error Detection and Improvement: Interpretability enables the identification of errors or biases in generative AI systems. When developers and users can understand the decision-making process, they can identify problematic patterns or outputs and make necessary improvements. Interpretability empowers stakeholders to address issues, refine the algorithms, and enhance the overall performance and reliability of the system.
- Supporting User Understanding and Control: Interpretability provides users with insights into how generative AI systems arrive at their outputs. This understanding empowers users to have more control over the generated content, allowing them to intervene or customize the outputs based on their preferences. User understanding and control foster a sense of ownership and enable more personalized and user-centric experiences.
- Ethical and Responsible AI Development: Evaluating fairness and interpretability aligns with the principles of ethical and responsible AI development. It ensures that generative AI systems are developed and used in a manner that respects fundamental rights, promotes fairness, and minimizes potential harm. Incorporating fairness and interpretability considerations into the design process demonstrates a commitment to ethical practices.
By prioritizing fairness and interpretability in generative AI systems, we can harness the full potential of these technologies while mitigating risks and maximizing benefits. Fairness evaluation and interpretability contribute to user trust, accountability, error detection, user understanding, and responsible AI development. Ultimately, this fosters the development and deployment of generative AI systems that are more aligned with societal values, promoting fairness, inclusivity, and the ethical use of AI in various domains.
Conclusion
In conclusion, ensuring the fairness and interpretability of generative AI systems is crucial for their responsible and ethical use. By addressing biases, promoting transparency, and enabling user understanding, we can reap several benefits in deploying these systems.
Through fairness evaluation, biases and discriminatory patterns can be identified and mitigated, fostering inclusivity and preventing harm to marginalized groups. This promotes a more equitable and diverse generation of content. Additionally, by improving interpretability, we enhance user trust, acceptance, and engagement with generative AI systems. Users can better comprehend how the system operates and verify that it aligns with their expectations and values.
Fairness and interpretability also contribute to accountability and responsible AI use. They allow stakeholders to identify errors, biases, or unintended consequences and take appropriate measures to rectify them. This leads to continuous improvement and refinement of generative AI systems, ensuring that they align with ethical guidelines and legal frameworks.
Moreover, the focus on fairness and interpretability supports user understanding and control. Users can gain insights into the decision-making process and customize or intervene in the generated outputs, empowering them to shape their experiences and content according to their preferences.
By integrating fairness and interpretability into the development and evaluation of generative AI systems, we foster a culture of responsible AI use. This not only builds trust and acceptance among users but also aligns with ethical principles and societal values. It ensures that generative AI technology is harnessed to benefit individuals and communities without perpetuating biases or harm.
Moving forward, it is essential for researchers, developers, and policymakers to continue advancing the methodologies and frameworks for evaluating fairness and interpretability in generative AI systems. By doing so, we can unlock the full potential of these systems in various domains, while upholding fairness, transparency, and accountability in the deployment and use of AI technologies.
Post a Comment