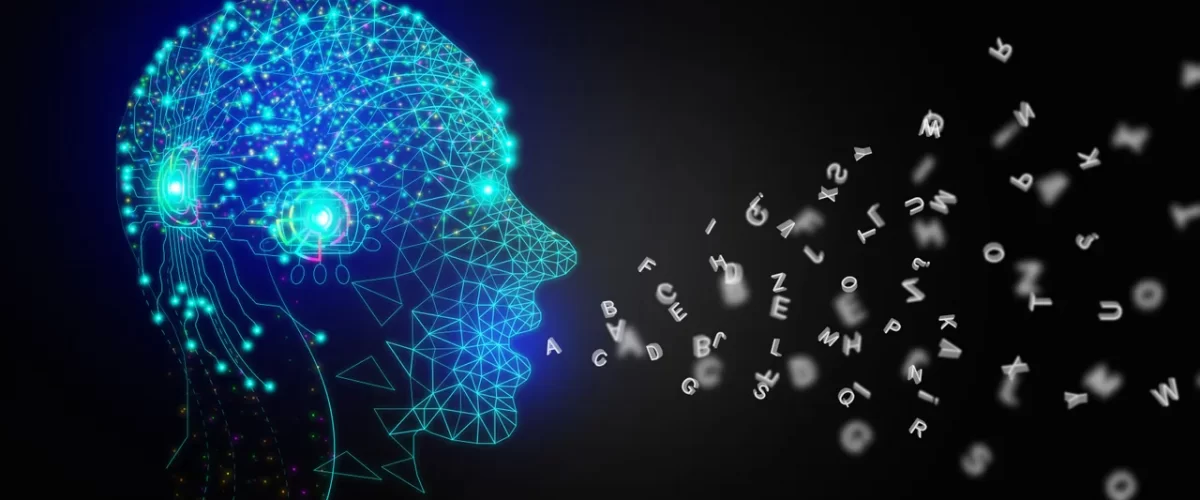
Generative Artificial Intelligence (AI) models have revolutionized various domains, from natural language processing and computer vision to music composition and image synthesis. These models, such as generative adversarial networks (GANs) and autoregressive models like transformers, have demonstrated impressive capabilities in generating realistic and coherent content, opening up exciting possibilities in creative fields and problem-solving applications. However, despite their remarkable achievements, generative AI models face a set of challenges and limitations that researchers and developers must address to further enhance their effectiveness and reliability.
This article aims to explore some of the key challenges and limitations associated with generative AI models. By understanding these constraints, we can gain insights into the current state of the technology and identify areas for future advancements.
Challenges of Generative AI Models
Generative AI models, while powerful and transformative, face several significant challenges. These challenges include:
- Data Availability and Quality: Generative AI models heavily rely on large and diverse datasets for training. However, acquiring such datasets can be a challenge. Collecting and curating high-quality data that adequately represents the target distribution can be time-consuming, expensive, and subject to biases. In many cases, limited or insufficient data may be available, making it difficult to train models effectively.
- Mode Collapse and Lack of Diversity: Mode collapse occurs when a generative model fails to capture the full diversity of the training data and instead produces limited or repetitive outputs. This limitation is especially prominent in generative adversarial networks (GANs), where the generator may converge to a subset of the target distribution, neglecting other possibilities. Achieving diversity and avoiding mode collapse remains an active area of research.
- Interpretability and Control: Generative AI models often lack interpretability, making it challenging to understand how they generate outputs or make decisions. The complex architectures and algorithms used in these models make it difficult to trace the reasoning behind their outputs. This lack of interpretability raises concerns in critical applications where transparency, explanations, and control over the generated content are essential.
- Ethical and Biased Outputs: Generative AI models can inadvertently learn biases present in the training data. If the training data contains biases, the model may generate outputs that reflect and amplify those biases. Ensuring fairness, avoiding discrimination, and addressing ethical concerns in generative AI models are important considerations that need to be actively addressed.
- Uncertainty and Risk Management: Generative models often struggle with quantifying uncertainty and managing risk. Assessing the reliability and confidence of generated outputs is crucial, especially when these models are deployed in real-world applications. Understanding and accurately characterizing the uncertainty associated with generative AI models is an ongoing challenge that needs to be tackled.
- Computational Resources and Efficiency: Training and deploying large-scale generative AI models require significant computational resources. The complex architectures and massive datasets used in these models demand high-performance hardware and substantial memory. Scaling up and optimizing the training and inference processes to improve efficiency and reduce resource requirements are important challenges that need to be addressed for wider adoption.
- Generalization and Robustness: Generative AI models often struggle with generalizing to unseen data or handling input variations. They may generate unrealistic or inconsistent outputs when presented with inputs that deviate from the training distribution. Ensuring robustness and generalization capabilities across diverse scenarios and inputs remains a challenge in the field of generative AI.
By addressing these challenges, researchers and developers can enhance the effectiveness, reliability, and ethical implications of generative AI models. Overcoming these limitations will open up new opportunities for the application of generative AI in various domains, leading to more responsible and impactful deployment of these models.
Limitations of Generative AI Models
Generative AI models, despite their impressive capabilities, have several limitations that impact their performance and application. These limitations include:
- Training Data Requirements: Generative AI models typically require large amounts of high-quality training data to learn and generate outputs effectively. Obtaining such data can be challenging, especially in domains where labeled or diverse datasets are scarce. Insufficient or biased training data can result in poor quality or biased generated content.
- Mode Collapse and Lack of Diversity: Mode collapse refers to a situation where a generative model fails to capture the full diversity of the target distribution and instead generates limited or repetitive outputs. This limitation is particularly evident in generative adversarial networks (GANs), where the generator may converge to a subset of the target distribution, ignoring other modes. Achieving diverse and realistic outputs across the entire distribution remains a challenge.
- Lack of Fine-Grained Control: Generative AI models often lack fine-grained control over the generated outputs. While they can generate novel and creative content, controlling specific attributes or characteristics of the output can be challenging. This limitation restricts their utility in applications that require precise customization or control over the generated content.
- Interpretability and Explainability: Generative AI models are often considered black boxes, making it difficult to understand their decision-making processes or provide explanations for the generated outputs. The complex architectures and intricate interactions within the models hinder interpretability. This lack of transparency can limit their adoption in critical domains where explainability is crucial.
- Uncertainty and Risk Management: Generative AI models typically struggle with quantifying and managing uncertainty in their outputs. Assessing the reliability and confidence of generated content becomes challenging, which can hinder their deployment in safety-critical applications. Addressing uncertainty estimation and managing associated risks are ongoing research areas.
- Ethical Considerations and Bias: Generative AI models can inadvertently inherent biases present in the training data, leading to the generation of biased or unfair content. Ensuring fairness, mitigating biases, and avoiding the generation of inappropriate or offensive content are significant challenges. Ethical considerations and responsible use of generative AI models are crucial for their widespread acceptance.
- Computationally Demanding: Training and deploying generative AI models can be computationally intensive and resource-demanding. Large-scale models with complex architectures require powerful hardware and substantial memory. This limits the accessibility and practicality of using generative AI models in resource-constrained environments or for real-time applications.
- Generalization to Unseen Data: Generative AI models may struggle to generalize well to unseen data or variations outside the training distribution. They may generate unrealistic or inconsistent outputs when presented with inputs that differ significantly from the training data. Ensuring robust generalization capabilities across diverse scenarios and inputs remains a challenge.
Understanding these limitations is crucial for researchers, developers, and users of generative AI models. Addressing these challenges through ongoing research and innovation will contribute to improving the capabilities, reliability, and ethical implications of generative AI models, enabling their broader adoption in various domains.
Conclusion
In conclusion, generative AI models have demonstrated remarkable capabilities in generating content across various domains. However, they face several challenges and limitations that researchers and developers must address to enhance their effectiveness, reliability, and ethical implications.
The challenges include acquiring high-quality and diverse training data, avoiding mode collapse to achieve diversity in generated outputs, ensuring interpretability and control over the models, managing uncertainty and quantifying risk, optimizing computational resources and efficiency, and addressing ethical concerns such as bias and inappropriate content generation. Additionally, the ability of generative AI models to generalize to unseen data and handle input variations is an ongoing challenge.
By actively tackling these challenges, researchers and developers can improve the performance and application of generative AI models. This will lead to more responsible and impactful deployment of these models in various domains, fostering creativity, problem-solving, and innovation. The continuous advancements in addressing these limitations will unlock the full potential of generative AI models, enabling them to reshape industries and contribute to the development of society.
Post a Comment