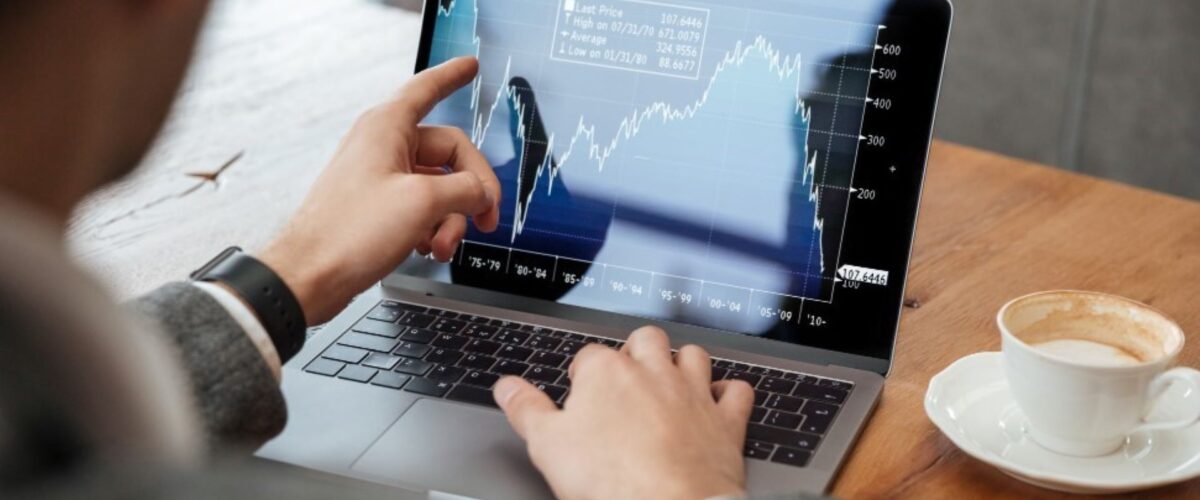
Applying generative AI techniques to financial forecasting and stock market analysis has emerged as a promising field, offering new perspectives and insights into the complex dynamics of financial markets. By leveraging advanced generative models, such as deep learning and recurrent neural networks, it becomes possible to analyze historical data, detect patterns, and generate forecasts to aid in decision-making processes.
Financial forecasting involves predicting future trends, movements, and values of financial assets, while stock market analysis focuses specifically on understanding the dynamics of stock prices and identifying potential investment opportunities. Traditional methods of financial analysis often rely on statistical models and fundamental analysis techniques. However, generative AI introduces a new dimension by incorporating the power of machine learning and pattern recognition into the process.
Generative AI models can learn from historical financial data, capturing underlying patterns and relationships between various market factors. These models can identify complex dependencies, nonlinear relationships, and temporal dynamics that are challenging to capture using traditional approaches. By analyzing large volumes of financial data, including stock prices, trading volumes, economic indicators, news sentiment, and more, generative AI models can uncover hidden patterns and generate forecasts with the potential for enhanced accuracy.
Benefits of Generative AI to Financial Forecasting and Stock Market Analysis
The application of generative AI to financial forecasting and stock market analysis offers several potential benefits:
- Improved Prediction Accuracy: Generative AI models can potentially offer improved prediction accuracy by leveraging the power of deep learning and pattern recognition. These models can identify intricate market dynamics and capture subtle patterns that may not be evident using traditional methods, leading to more accurate forecasts.
- Real-time Analysis and Decision-making: Generative AI models can analyze vast amounts of financial data in real-time, enabling timely decision-making. This is particularly valuable in fast-paced financial markets where rapid responses and insights can be critical for investors and traders.
- Identification of Complex Market Patterns: Generative AI techniques can uncover complex patterns and relationships in financial data that may not be apparent to human analysts. These models can detect non-linear dependencies, correlations, and anomalies, allowing for a deeper understanding of market dynamics and potential investment opportunities.
- Risk Assessment and Management: By analyzing historical data and generating forecasts, generative AI models can assist in assessing and managing financial risks. These models can provide insights into market volatility, identify potential market crashes or bubble formations, and support risk mitigation strategies.
- Portfolio Optimization: Generative AI techniques can aid in optimizing investment portfolios by suggesting allocation strategies based on forecasted market trends. These models can help investors make informed decisions about diversification, asset allocation, and risk-return trade-offs.
- Automation and Scalability: Generative AI can automate various aspects of financial analysis and forecasting, allowing for scalability and efficiency. By reducing manual effort in data analysis and prediction generation, financial professionals can focus on higher-level tasks, such as strategy development and risk management.
The application of generative AI to financial forecasting and stock market analysis is an evolving field with immense potential. However, it is important to note that financial markets are influenced by numerous factors, including economic conditions, geopolitical events, and investor sentiment, which may introduce complexities and uncertainties. Careful validation, continuous model improvement, and human expertise remain essential for reliable and responsible decision-making in the financial domain.
Conclusion
In conclusion, the application of generative AI techniques to financial forecasting and stock market analysis holds significant promise for enhancing decision-making processes in the realm of finance. By leveraging advanced generative models, such as deep learning and recurrent neural networks, it becomes possible to analyze vast amounts of historical financial data, detect patterns, and generate forecasts with the potential for improved accuracy.
The benefits of applying generative AI to financial forecasting and stock market analysis are manifold. These techniques offer the potential for improved prediction accuracy by capturing complex market dynamics and uncovering subtle patterns that may not be evident using traditional approaches. Real-time analysis and decision-making are facilitated by the ability of generative AI models to process large volumes of financial data rapidly.
Generative AI models excel at identifying complex market patterns, including non-linear relationships, dependencies, and anomalies. This enables a deeper understanding of market dynamics and provides insights into potential investment opportunities. Additionally, generative AI models can contribute to risk assessment and management by analyzing historical data and offering insights into market volatility, potential crashes, or bubble formations.
Furthermore, the application of generative AI can support portfolio optimization by suggesting allocation strategies based on forecasted market trends. Automation and scalability are additional benefits, as generative AI techniques automate various aspects of financial analysis and forecasting, allowing financial professionals to focus on higher-level tasks.
It is important to note that financial markets are influenced by a multitude of factors, including economic conditions and geopolitical events, introducing complexities and uncertainties. Thus, continuous model validation, improvement, and the incorporation of human expertise remain crucial for reliable and responsible decision-making in the financial domain.
As the field of generative AI continues to advance, further research and development in applying these techniques to financial forecasting and stock market analysis can contribute to more accurate predictions, improved risk management, and enhanced decision-making in the financial industry.
Post a Comment