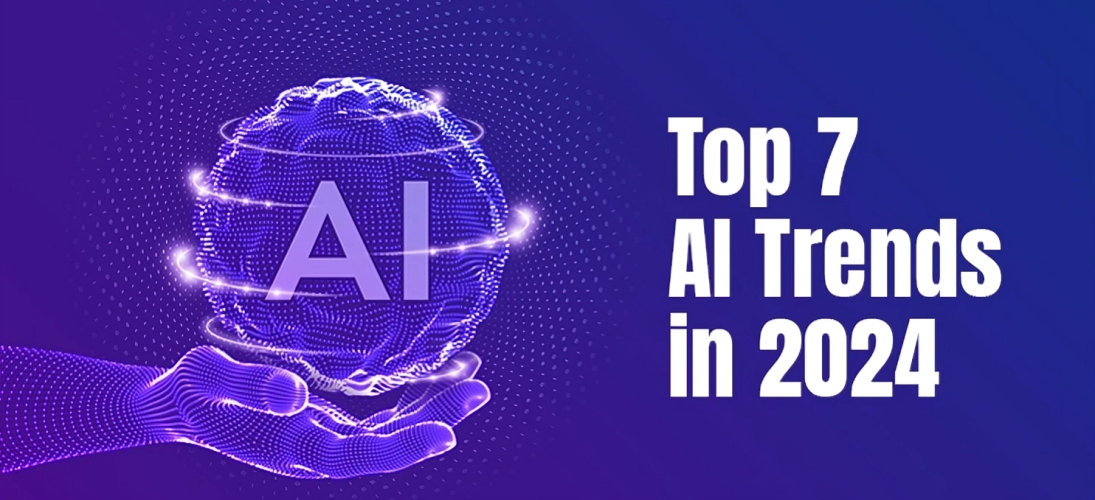
1.Multimodal AI
While traditional single-mode data processing involves text, images, and sound, multimodal AI goes beyond that by hosting these different input types, one step closer to emulating the human capacity to process a variety of senses.
“The interfaces of the world are multimodal,” said Mark Chen, head of frontiers research at OpenAI, in a presentation at the conference EmTech MIT in November 2023. “We want our models to see what we see and hear what we hear, and we want them to also generate content that appeals to more than one of our senses”.
2. Agentic Ai
Agentic AI represents the significant leap from Reactive to Proactive AI. Advanced systems that show aspects of autonomy, proactivity, and the ability to act independently are considered AI agents. Unlike traditional AI systems, which for the most part reactively respond to inputs by users and follow the programming installed in them, AI agents are designed to perceive their environment, establish goals, and act towards achieving those objectives without direct human intervention.
This would mean that an AI agent could be engaged in environmental monitoring for data acquisition, pattern analyses, and the initiation of preventive actions relative to hazards, such as an early warning against forest fire. An AI agent could also be used in finance to actively manage an investment portfolio by means of adaptive strategies.
3. Open source Ai
It is a deeply expensive process to build large language models and other generative AI systems, which requires exorbitant amounts of compute and data. Using an open source model, however, allows the developer to build on top of others’ work, reducing the costs and expanding access to AI more generally. Open-source AI publicly makes source code available for organizations and researchers to contribute to and build off existing code.
That is evident in GitHub data from the past year: developer engagement with the tech, in particular generative AI, has grown remarkably. For the first time, generative AI projects broke into the top 10 most popular projects across the code hosting platform in 2024.
4.Retrieval-augmented generation
While generative AI tools saw wide adoption in 2023, they remain afflicted by one of the same limitations: halluci nations, or plausible-sounding but wrong answers to user queries. The limitation has been a roadblock to enterprise adoption, as hallucinations in business-critical or customer-facing scenarios could be catastrophic.
One technique for reducing hallucinations emerged under the name Retrieval-augmented generation, or RAG; the implications for enterprise AI adoption could be profound.
RAG brings together the power of text generation with information retrieval to increase the accuracy and relevance of the AI-generated content.
5.Customized enterprise generative AI models
To date, consumer interest in generative AI has centered around massive, general-purpose tools like Midjourney and ChatGPT. But when it comes to business use cases, it may be the smaller, narrow-purpose models that have the most staying power as demand grows for more workable AI systems that can meet niche requirements.
While that’s theoretically possible, creating a new model from scratch is such a resource-intensive proposition that most organizations won’t be able to afford the time, talent, and compute resources required to build and train one. Most organizations don’t build generative AI from scratch but tune an existing AI model, for instance, by tweaking their architecture or fine tuning on a domain-specific data set.
6.Need for AI and machine learning talent
Designing, training, and testing a machine learning model-not to mention pushing it into production and maintaining it in a complex organizational IT environment-is by no means an easy task. No wonder, therefore, this growing need for AI and machine learning talent is expected to continue well into 2024 and beyond.
“The market is still really hot around talent,” Luke said. “It’s very easy to get a job in this space.”
Concretely, while artificial intelligence and machine learning are becoming part of every business, there is an increasing requirement for experts who can bridge the gap between theory and practice.
7. Shadow AI
But as workers of all types begin to take notice of generative AI, organizations must contend with the growing problem of shadow AI-the use of AI inside an organization without explicit approval or oversight from IT. Indeed, it is fast becoming a trend as AI becomes increasingly accessible to allow even non-technical workers to use it independently.
Shadow AI usually comes about when employees need quick fixes for a problem or want to try out new technology faster than the official channels will allow. This is especially the case with easy-to-use AI chatbots, where, for example, testing can be done in employees’ web browsers without too much difficulty, rather than having to go through IT review and approval processes.
- September 4, 2024
- By:A A
- Categories:Uncategorized
- Tags: AI, Generative AI, Machine Learning
- no comments
Post a Comment