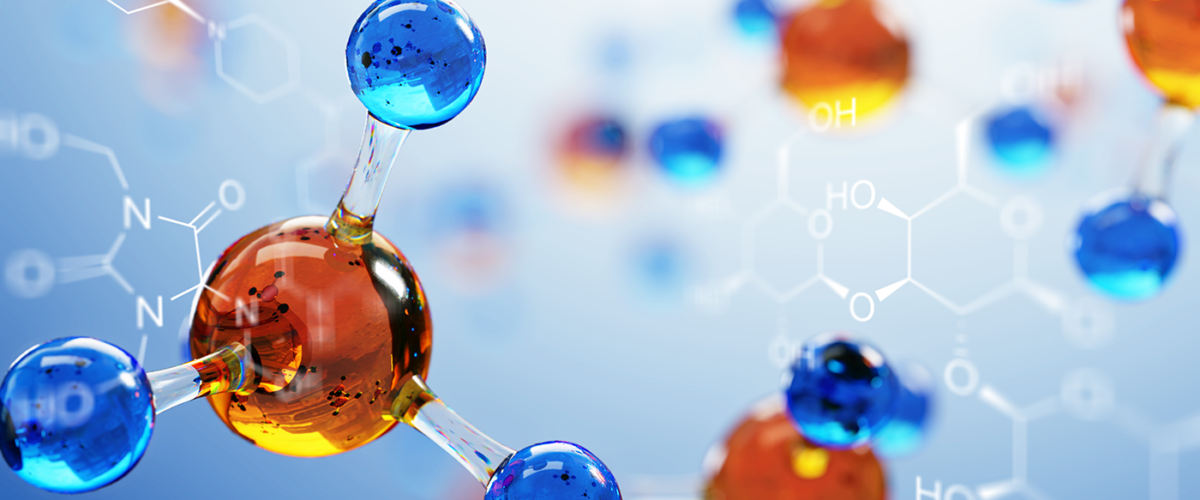
In recent years, the field of drug discovery and molecular design has witnessed a transformative shift with the advent of generative artificial intelligence (AI) technologies. Traditional drug discovery approaches heavily rely on laborious experimentation and trial-and-error processes, often resulting in high costs, lengthy timelines, and limited success rates. However, with the integration of generative AI models, researchers now have powerful tools at their disposal to expedite the discovery and design of novel drugs with improved efficacy and reduced side effects.
Generative AI refers to a subset of artificial intelligence that focuses on creating new and original content. In the context of drug discovery and molecular design, generative AI algorithms harness the power of deep learning and machine learning techniques to generate virtual molecules, predict their properties, and optimize their structures to meet specific therapeutic targets. These algorithms learn from vast amounts of existing data, including chemical databases, biological assays, and molecular interactions, enabling them to make predictions and propose new molecules with desired characteristics.
The impact of generative AI in drug discovery and molecular design has been profound. One of the key advantages is the ability to accelerate the process of identifying lead compounds. By leveraging generative AI models, researchers can explore an enormous chemical space in a fraction of the time it would take using traditional methods. These models can generate vast libraries of virtual compounds that can be screened computationally, significantly increasing the chances of identifying promising candidates for further experimentation.
Moreover, generative AI algorithms can optimize the properties of generated molecules based on desired criteria, such as target specificity, potency, and safety profiles. This capability allows researchers to fine-tune molecules and make iterative improvements, enhancing the chances of discovering drug candidates with optimal efficacy and minimal side effects. By reducing the reliance on trial-and-error experimentation, generative AI expedites the drug discovery process, potentially saving years of research and development efforts.
Another significant impact of generative AI lies in its ability to facilitate the repurposing of existing drugs. With the vast amounts of available data, including information on approved drugs, failed candidates, and experimental compounds, generative AI models can explore potential new therapeutic applications for existing molecules. This repurposing approach can not only save time and resources but also provide new treatment options for diseases where traditional drug discovery efforts have fallen short.
Despite the tremendous promise of generative AI, challenges and limitations still exist. The generated molecules need to undergo rigorous experimental validation, and the models must continuously learn and adapt from new data to improve their accuracy and predictive capabilities. Ethical considerations, such as data privacy and responsible use of AI, also need to be addressed to ensure the technology’s responsible and equitable application in drug discovery.
In conclusion, the integration of generative AI in drug discovery and molecular design has revolutionized the field, enabling researchers to explore vast chemical spaces, accelerate lead identification, optimize molecular properties, and facilitate drug repurposing. By combining the power of deep learning algorithms with traditional experimental approaches, generative AI has the potential to reshape the pharmaceutical industry, bringing innovative and effective therapies to patients faster and more efficiently than ever before.
Advantages of Generative AI in Drug Discovery and Molecular Design
Generative AI in drug discovery and molecular design offers several advantages that have significantly impacted the field. Some of the key advantages include:
- Accelerated Lead Identification: Generative AI algorithms can rapidly generate and screen vast libraries of virtual compounds, allowing researchers to explore a much larger chemical space compared to traditional methods. This acceleration in lead identification expedites the early stages of drug discovery and increases the likelihood of finding promising candidates for further experimentation.
- Exploration of Novel Chemical Space: Generative AI models can generate molecules that may have never been synthesized or tested before. This enables researchers to explore uncharted areas of chemical space, potentially leading to the discovery of new molecular scaffolds and therapeutic targets that were previously overlooked. It opens up possibilities for innovative drug design and the development of novel treatments.
- Optimization of Molecular Properties: Generative AI algorithms can optimize the properties of generated molecules based on specific criteria, such as potency, selectivity, solubility, and safety. Through iterative improvements, these models can propose molecules with enhanced efficacy and reduced side effects, saving time and resources compared to traditional trial-and-error experimentation.
- Repurposing of Existing Drugs: Generative AI can assist in identifying alternative therapeutic applications for existing drugs. By leveraging large databases of drug-related information, AI models can suggest potential new uses for approved drugs, failed candidates, or experimental compounds. This repurposing approach offers a cost-effective strategy to discover new indications and expand the clinical utility of existing medications.
- Efficient Resource Utilization: Traditional drug discovery involves extensive experimental work, which can be time-consuming and expensive. Generative AI reduces the need for exhaustive laboratory synthesis and testing by prioritizing and selecting the most promising molecules for further evaluation. This streamlines the drug discovery process, making it more efficient and cost-effective.
- Rapid Iterative Design: Generative AI allows for quick iterations and modifications of molecular structures. Researchers can generate variations of a molecule and assess their properties in a computationally efficient manner. This capability enables researchers to fine-tune and optimize molecules in a much shorter time frame compared to traditional approaches.
- Data-Driven Decision Making: Generative AI models are trained on vast amounts of chemical and biological data, including information from public and proprietary databases. By learning from this data, AI algorithms can make data-driven predictions and propose molecules with desired properties. This reduces reliance on subjective decision-making and enhances the likelihood of successful drug discovery outcomes.
- Enhanced Collaboration and Knowledge Sharing: Generative AI facilitates the sharing of knowledge and collaboration among researchers. AI models can be trained on diverse datasets, incorporating insights and findings from multiple sources. This collective intelligence improves the overall quality of the models and promotes collaboration within the scientific community.
Disadvantages of Generative AI in Drug Discovery and Molecular Design
While generative AI in drug discovery and molecular design offers numerous advantages, it also has some limitations and potential disadvantages that need to be considered. These disadvantages include:
- Limited Chemical Diversity: Generative AI models are trained on existing data, which may not capture the full spectrum of chemical diversity. As a result, there is a risk that the generated molecules may resemble existing compounds rather than exploring entirely new chemical space. This limitation can hinder the discovery of truly innovative and unique molecular structures.
- Lack of Experimental Validation: The molecules generated by AI models require experimental validation to confirm their properties and biological activities. While AI can suggest potential candidates, laboratory synthesis and testing are still necessary to assess their efficacy, safety, and pharmacokinetic properties. This validation process can be time-consuming and resource-intensive, potentially offsetting the initial time savings provided by generative AI.
- Uncertainty and Interpretability: Generative AI models often operate as black boxes, meaning that the decision-making process and reasoning behind generated molecules may not be easily interpretable. This lack of transparency and interpretability can be a challenge for researchers who need to understand the underlying principles driving the generated results and make informed decisions based on them.
- Biased Training Data: AI models heavily rely on the quality and representativeness of the training data. If the training data is biased or incomplete, the generated molecules may inherit these biases, leading to potential limitations in the diversity and applicability of the generated compounds. It is crucial to address and mitigate biases in training data to ensure the fairness and inclusivity of AI-driven drug discovery efforts.
- Ethical Considerations: The use of generative AI in drug discovery raises ethical concerns that need to be carefully addressed. These include data privacy, ownership and sharing of proprietary data, responsible use of AI, and potential implications for patient privacy and consent. Ensuring ethical and responsible practices in the use of generative AI is essential to maintain trust and uphold ethical standards in the field.
- Over Reliance on Computational Models: While generative AI provides powerful tools for virtual screening and optimization, it should not completely replace experimental methods. Experimental validation is still crucial for confirming the properties and behavior of generated molecules. Over Reliance on computational models without sufficient experimental validation can lead to false positives or false negatives, potentially compromising the reliability of the drug discovery process.
- High Computational Resource Requirements: Training and running generative AI models can be computationally demanding, requiring significant computational resources and infrastructure. This can be a barrier for smaller research groups or organizations with limited access to high-performance computing, limiting their ability to fully leverage generative AI technologies in drug discovery.
- Regulatory Challenges: The integration of generative AI in drug discovery raises regulatory challenges, particularly in the context of intellectual property and regulatory approval processes. The unique nature of AI-generated molecules and their potential classification under existing regulatory frameworks may require new guidelines and adaptations to ensure compliance and facilitate the translation of AI-driven discoveries into clinical applications.
In conclusion, while generative AI offers great potential in drug discovery and molecular design, there are several limitations and potential disadvantages to consider. Addressing these challenges requires careful attention to ensure the reliability, interpretability, and ethical use of generative AI technologies in the context of drug discovery. By understanding and mitigating these limitations, researchers can harness the power of generative AI to accelerate and enhance the drug discovery process.
Post a Comment