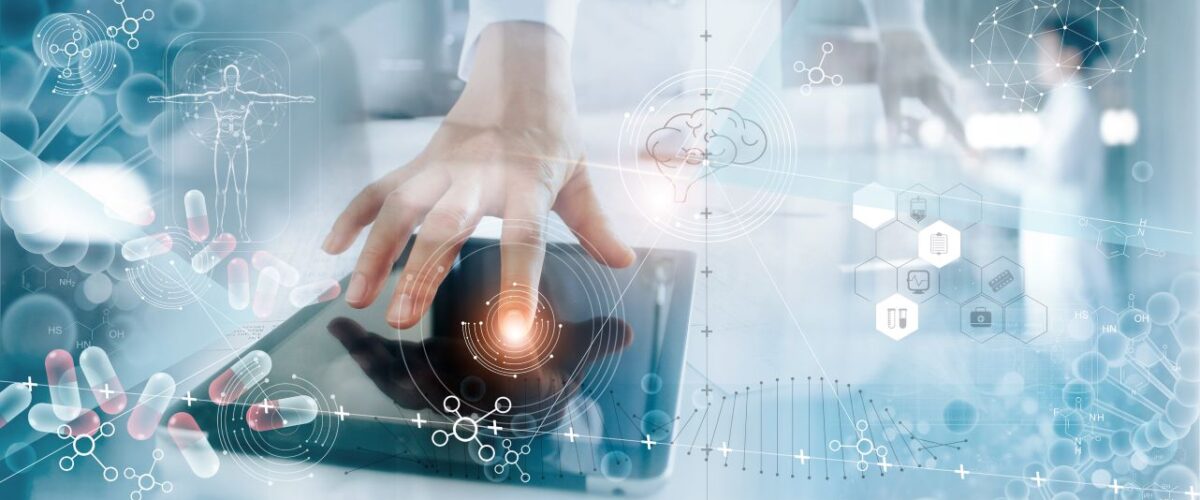
Medical imaging plays a crucial role in diagnosing and monitoring various diseases and conditions. Traditional medical imaging techniques, such as X-ray, computed tomography (CT), magnetic resonance imaging (MRI), and ultrasound, generate detailed visual representations of the human body’s internal structures. However, the interpretation of these images and the extraction of meaningful information often rely on the expertise of trained radiologists and clinicians.
Generative artificial intelligence (AI) has emerged as a transformative technology in the field of medical imaging. By leveraging deep learning and machine learning algorithms, generative AI models can analyze vast amounts of medical image data, learn patterns, and generate new images that are realistic and representative of specific medical conditions. This integration of generative AI in medical imaging has the potential to revolutionize diagnostics, enhance treatment planning, and advance research in the field of healthcare.
Generative AI models in medical imaging can generate synthetic images that closely resemble real patient data. These models are trained on large datasets containing labeled medical images, enabling them to learn the underlying patterns and characteristics of different diseases or anatomical structures. Once trained, these models can generate new images with variations, providing insights into how specific conditions might manifest and aiding in the identification of subtle abnormalities.
The impact of generative AI in medical imaging is far-reaching. It offers several advantages, such as improved accuracy in detecting and diagnosing diseases, enhanced visualization of complex anatomical structures, and personalized treatment planning. Additionally, generative AI can assist in image reconstruction, noise reduction, and data augmentation, leading to better image quality and reducing the need for additional imaging procedures.
Furthermore, generative AI models can aid in the development of new imaging techniques and protocols. By simulating realistic medical images, researchers can test and refine imaging algorithms, assess the performance of novel imaging modalities, and optimize imaging parameters without exposing patients to unnecessary risks or radiation.
Despite the significant potential of generative AI in medical imaging, challenges remain. The ethical use of patient data, privacy concerns, and the need for transparent and interpretable AI algorithms are critical considerations. Additionally, ensuring the robustness, generalizability, and reliability of generative AI models in diverse patient populations is crucial for their successful integration into clinical practice.
In conclusion, generative AI is poised to transform medical imaging by enabling the generation of realistic and informative images that aid in disease detection, diagnosis, treatment planning, and research. As this technology continues to advance, it has the potential to augment the capabilities of healthcare professionals, improve patient outcomes, and accelerate advancements in medical imaging, ultimately leading to more precise and personalized healthcare interventions.
Benefits of Enhancing Diagnostics and Research in Generative AI
Enhancing diagnostics and research through the application of generative AI in healthcare and medical imaging offers several notable benefits. These benefits include:
- Improved Accuracy and Precision: Generative AI models have the potential to enhance diagnostic accuracy and precision by assisting healthcare professionals in interpreting medical images. These models can identify subtle patterns, anomalies, and early-stage disease indicators that might be challenging for human observers to detect. By providing additional insights and reducing diagnostic errors, generative AI can improve patient outcomes and optimize treatment strategies.
- Early Detection and Diagnosis: Generative AI models can help identify disease markers and patterns at early stages when they may not be easily distinguishable by traditional imaging techniques. By detecting diseases and conditions earlier, clinicians can intervene sooner, leading to better prognosis and increased chances of successful treatment.
- Personalized Medicine: Generative AI can aid in the development of personalized treatment plans by analyzing medical images and generating patient-specific models. These models can simulate the progression of diseases, predict treatment outcomes, and enable clinicians to tailor interventions based on individual patient characteristics. This approach has the potential to optimize therapy selection, dosage determination, and treatment monitoring, leading to improved patient responses and reduced adverse effects.
- Enhanced Visualization and Interpretation: Generative AI can generate synthetic medical images that provide clearer and more detailed visualizations of anatomical structures, tissues, and abnormalities. These enhanced visualizations can assist healthcare professionals in better understanding complex structures and identifying subtle features that might be challenging to discern in traditional imaging. Improved visualization aids in accurate interpretation and decision-making, ultimately benefiting patient management.
- Data Augmentation and Reconstruction: Generative AI can be used to augment existing medical image datasets, increasing their diversity and size. By synthesizing additional images, AI models can train on more comprehensive data, improving their performance and generalizability. Additionally, generative AI can reconstruct low-quality or noisy images, enhancing their quality and facilitating more accurate diagnoses and analyses.
- Facilitating Research and Clinical Trials: Generative AI models can generate synthetic datasets that mimic real-world patient populations, enabling researchers to conduct simulations, test algorithms, and validate hypotheses without directly involving human subjects. This capability expedites the research process, enhances data availability, and facilitates the design and evaluation of clinical trials.
- Novel Imaging Technique Development: Generative AI can aid in the development of new imaging techniques and protocols. By generating realistic medical images, researchers can simulate and optimize imaging parameters, assess the performance of novel imaging modalities, and test innovative image reconstruction algorithms. This iterative process accelerates the development of advanced imaging technologies, enabling earlier clinical adoption and improving patient care.
- Cost and Time Efficiency: Generative AI can potentially reduce healthcare costs and imaging time by optimizing imaging protocols, reducing the need for unnecessary imaging procedures, and improving the efficiency of image interpretation. This benefit not only improves resource utilization but also expedites patient diagnosis and treatment, leading to faster and more efficient healthcare delivery.
Disadvantages of Enhancing Diagnostics and Research in Generative AI
While enhancing diagnostics and research through generative AI in healthcare and medical imaging offers numerous advantages, there are also several potential disadvantages and challenges that need to be considered. These disadvantages include:
- Ethical and Privacy Concerns: The use of generative AI in healthcare raises ethical considerations regarding patient privacy, data security, and consent. The generation and analysis of medical images involve sensitive patient data, and ensuring proper data anonymization and protection is crucial to maintain patient confidentiality and trust.
- Interpretability and Explainability: Generative AI models often operate as black boxes, making it challenging to interpret and explain the reasoning behind their decisions. The lack of interpretability can be a significant limitation in healthcare, where understanding the model’s decision-making process is crucial for clinical acceptance and trust.
- Generalization to Diverse Populations: Generative AI models need to be trained on diverse and representative datasets to ensure their performance across different patient populations. Failure to capture the full range of variations and demographic factors may result in biased or inaccurate predictions, limiting the model’s generalizability and effectiveness.
- Lack of Real-world Validation: Although generative AI models can produce realistic synthetic images, they still need to be validated with real-world clinical data. The reliance on simulated or generated data without extensive validation using actual patient outcomes and longitudinal studies can introduce uncertainty and potential discrepancies between the model’s performance and real-world effectiveness.
- Resource Requirements and Computational Complexity: Training and deploying generative AI models often require significant computational resources, including high-performance computing infrastructure and large-scale datasets. The computational complexity and resource requirements can limit the accessibility and widespread adoption of generative AI technology, particularly in resource-constrained healthcare settings.
- Limited Availability of Labeled Data: Generative AI models rely on large, labeled datasets for training. However, obtaining and labeling medical imaging data can be a time-consuming and labor-intensive process. The limited availability of annotated datasets, particularly for rare diseases or specific populations, can hinder the development and performance of generative AI models in certain areas of medical imaging.
- Regulatory and Legal Considerations: The integration of generative AI in healthcare requires compliance with regulatory standards and legal frameworks. The use of AI in medical decision-making raises concerns about liability, accountability, and the need for regulatory approval. Clear guidelines and regulations are necessary to ensure safe and responsible deployment of generative AI in clinical practice.
- Overreliance and Human Expertise: While generative AI can assist healthcare professionals in diagnostics and research, it should not replace human expertise and clinical judgment. AI models should be considered as tools that support decision-making rather than making autonomous decisions. Healthcare professionals need to interpret the generated results in the context of clinical knowledge and patient-specific factors.
In conclusion, while generative AI has the potential to enhance diagnostics and research in healthcare and medical imaging, it is important to address the potential disadvantages and challenges associated with its implementation. Ethical considerations, interpretability, generalizability, real-world validation, resource requirements, data availability, regulatory compliance, and the role of human expertise are crucial factors that need to be carefully considered to ensure the responsible and effective integration of generative AI in healthcare.
Post a Comment