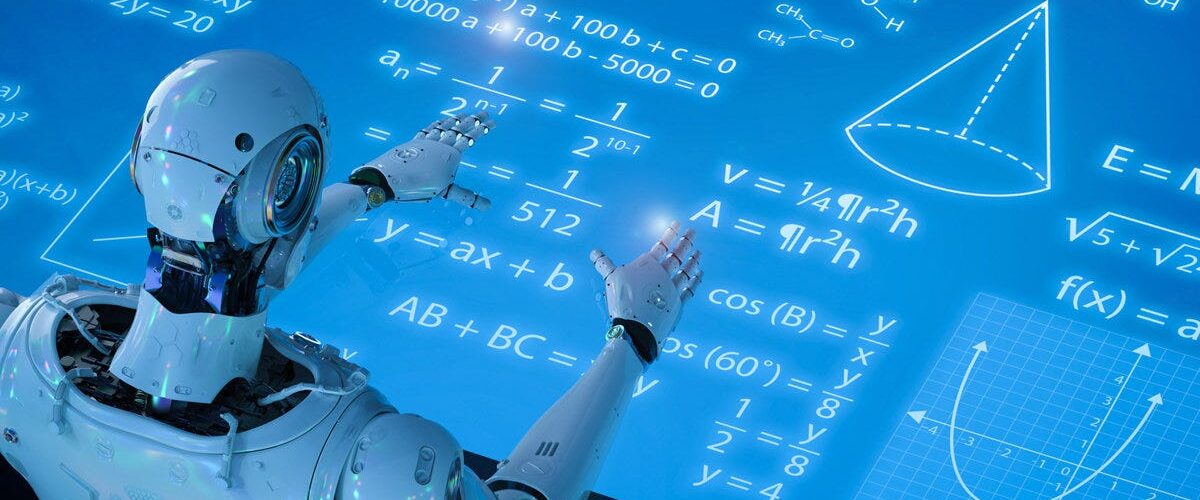
Generative AI has found applications in various domains, and one particularly critical area where it has shown significant promise is in the field of cybersecurity. With the ever-increasing sophistication and complexity of cyber threats, traditional rule-based and signature-based approaches alone are insufficient to combat emerging attacks. Generative AI offers a new paradigm that can enhance cybersecurity defenses by enabling proactive and adaptive measures to counteract evolving threats.
Generative AI in cybersecurity refers to the application of generative models, such as deep neural networks and variational autoencoders, to address various challenges in detecting, preventing, and mitigating cyber attacks. These models have the ability to learn patterns, behaviors, and anomalies from vast amounts of data, enabling them to identify and respond to threats in real-time.
By leveraging generative AI techniques, cybersecurity systems can perform tasks such as anomaly detection, threat intelligence analysis, malware detection, and attack generation and simulation. These models learn from historical attack data and legitimate network traffic to develop a deep understanding of normal and abnormal behaviors, thereby improving the accuracy of detection and reducing false positives.
Moreover, generative AI can also be utilized to create realistic and synthetic data, which can be used to train and test cybersecurity defenses. This approach helps in augmenting the limited availability of labeled datasets and enables the development of robust models capable of identifying new and unknown attack patterns.
In the face of adversarial attacks, where attackers deliberately manipulate or obfuscate data to evade detection, generative AI can assist in detecting and mitigating such threats. Adversarial training techniques leverage generative models to generate adversarial examples, which are then used to train defensive models to be more resilient and robust against sophisticated attacks.
However, the application of generative AI in cybersecurity is not without challenges. The need for large, diverse, and representative datasets for training robust models is a significant challenge in cybersecurity due to the limited availability of labeled data, privacy concerns, and legal restrictions. Additionally, ensuring the interpretability and explainability of generative AI models in cybersecurity is critical to building trust and understanding how decisions are made.
Despite these challenges, the integration of generative AI in cybersecurity holds immense potential. It enables organizations to stay ahead of the rapidly evolving threat landscape by proactively identifying and mitigating emerging attacks, augmenting human analysts’ capabilities, and enhancing the overall resilience and security of digital systems and networks. As research and development in this field continue to advance, generative AI in cybersecurity is expected to play a pivotal role in safeguarding our digital infrastructure and information assets.
Benefits of Enhancing Defense Mechanisms and Threat Detection
Enhancing defense mechanisms and threat detection in the field of cybersecurity brings several significant benefits. Here are some key advantages:
- Early Threat Detection: By leveraging advanced technologies like generative AI, cybersecurity systems can detect threats at an early stage, even before they can cause significant damage. Early detection allows for swift response and containment, minimizing the impact and potential loss associated with cyber attacks.
- Improved Accuracy: Generative AI models can learn complex patterns and behaviors from large datasets, enabling them to accurately identify anomalies and suspicious activities in real-time. This leads to reduced false positives and false negatives, enhancing the overall accuracy of threat detection systems.
- Proactive Defense: Traditional cybersecurity approaches often rely on predefined rules or signatures to identify known threats. However, generative AI can enable proactive defense by continuously learning from evolving attack patterns and adapting to new and previously unseen threats. This proactive approach helps organizations stay one step ahead of attackers.
- Adaptive and Dynamic Systems: Generative AI allows cybersecurity systems to adapt and evolve in response to changing attack techniques and strategies. These systems can learn from ongoing attacks, update their models, and improve their defenses, creating a dynamic and adaptive security environment.
- Enhanced Incident Response: Advanced threat detection mechanisms empower incident response teams with timely and accurate information about ongoing attacks. This enables quicker mitigation measures, reducing the time to detect, respond to, and recover from security incidents.
- Increased Efficiency: By automating certain aspects of threat detection and defense, generative AI technologies can help alleviate the burden on cybersecurity teams. This allows human analysts to focus on more complex and strategic tasks, improving overall operational efficiency.
- Defense Against Sophisticated Attacks: Cyber attackers are constantly developing new techniques and evading traditional security measures. Generative AI enhances defense mechanisms by identifying and countering advanced and targeted attacks that may go unnoticed by conventional methods.
- Scalability: Generative AI techniques, such as anomaly detection and behavior analysis, can be applied at scale across large networks and systems. This scalability enables efficient monitoring and protection of complex infrastructures, ensuring comprehensive security coverage.
- Reducing Dwell Time: Dwell time refers to the duration an attacker remains undetected within a system. By enhancing defense mechanisms and threat detection, organizations can reduce dwell time, minimizing the potential damage caused by persistent attackers and limiting their ability to move laterally across the network.
- Regulatory Compliance: Robust threat detection mechanisms contribute to meeting compliance requirements, particularly in industries with strict data protection and privacy regulations. Implementing effective defenses and demonstrating proactive threat detection can help organizations meet their legal and regulatory obligations.
By leveraging generative AI and advanced threat detection techniques, organizations can significantly strengthen their cybersecurity posture, improve incident response capabilities, and better protect their critical assets and sensitive information from evolving cyber threats.
Disadvantages of Enhancing Defense Mechanisms and Threat Detection
While enhancing defense mechanisms and threat detection in cybersecurity brings numerous benefits, there are also some disadvantages and challenges that organizations may encounter. Here are a few considerations:
- False Positives and False Negatives: Despite advancements in threat detection technologies, there is still a risk of false positives (classifying benign activities as threats) and false negatives (failing to identify actual threats). False positives can lead to unnecessary disruptions and false alarms, while false negatives can result in undetected attacks, leaving organizations vulnerable to potential breaches.
- High Costs and Resource Requirements: Implementing and maintaining advanced defense mechanisms and threat detection systems can be resource-intensive. It requires investment in specialized tools, hardware, software, and skilled personnel to operate and manage these systems effectively. Small organizations with limited resources may face challenges in implementing and sustaining such solutions.
- Complexity and Learning Curves: Advanced threat detection technologies, including generative AI, can be complex and require expertise to set up, configure, and fine-tune. Organizations may face a learning curve and require additional training or external assistance to maximize the effectiveness of these systems. Lack of in-house expertise can hinder the successful deployment and operation of advanced defense mechanisms.
- Privacy and Ethical Concerns: As defense mechanisms become more sophisticated, they may involve analyzing large amounts of data, including sensitive user information. This raises privacy concerns and ethical considerations, especially regarding data collection, storage, and usage. Organizations must carefully balance the need for effective defense with privacy protection and compliance with relevant regulations.
- Adversarial Attacks and Evolving Techniques: Cyber attackers continually develop new techniques and strategies to evade detection and circumvent defense mechanisms. Enhancing defense mechanisms may lead to a cat-and-mouse game, where attackers adapt their methods to exploit any weaknesses in the detection systems. This requires continuous monitoring, updating, and evolving defense mechanisms to stay ahead of evolving threats.
- User Acceptance and False Sense of Security: Introducing advanced defense mechanisms may impact user experience and productivity. Intrusive security measures or excessive false positives can lead to frustration among users, potentially leading to workarounds or resistance to security protocols. Additionally, organizations must ensure that users do not develop a false sense of security, assuming that advanced defenses can completely eliminate all risks.
- System Overhead and Performance Impact: Implementing sophisticated defense mechanisms may introduce additional system overhead and impact overall performance. Resource-intensive processes like deep packet inspection, real-time analysis, and machine learning algorithms can consume significant computing resources, potentially affecting network throughput, latency, and overall system performance.
- Evolving Threat Landscape: While advanced threat detection mechanisms can be effective against known threats, they may struggle with zero-day attacks or novel attack vectors. Attackers constantly innovate and exploit new vulnerabilities, making it challenging for defense mechanisms to keep pace. Continuous monitoring, research, and updates are required to address emerging threats effectively.
Organizations must carefully consider these disadvantages and challenges alongside the benefits when enhancing defense mechanisms and threat detection. Effective implementation and management, continuous monitoring, regular updates, and a holistic approach to cybersecurity are essential to mitigate these potential drawbacks and maximize the overall security posture.
Conclusion
Generative AI has emerged as a powerful tool in enhancing defense mechanisms and threat detection in the field of cybersecurity. It offers several benefits such as early threat detection, improved accuracy, proactive defense, adaptive systems, enhanced incident response, increased efficiency, defense against sophisticated attacks, scalability, and regulatory compliance.
However, organizations must also be aware of the potential disadvantages and challenges associated with these advancements. False positives and false negatives, high costs and resource requirements, complexity and learning curves, privacy and ethical concerns, adversarial attacks, user acceptance, system overhead, and the evolving threat landscape are factors that need to be carefully addressed.
To effectively leverage generative AI in cybersecurity, organizations must invest in the necessary resources, expertise, and ongoing training. They should strike a balance between security and user experience, ensuring privacy and ethical considerations are prioritized. Continuous monitoring, research, and updates are crucial to keep pace with the evolving threat landscape and maintain an effective defense.
Ultimately, generative AI has the potential to significantly strengthen cybersecurity defenses by providing proactive and adaptive measures, improving accuracy, and empowering organizations to stay ahead of emerging threats. With careful consideration of the challenges and diligent implementation, generative AI can play a pivotal role in safeguarding digital systems, protecting critical assets, and ensuring the resilience of organizations in the face of ever-evolving cyber threats.
Post a Comment