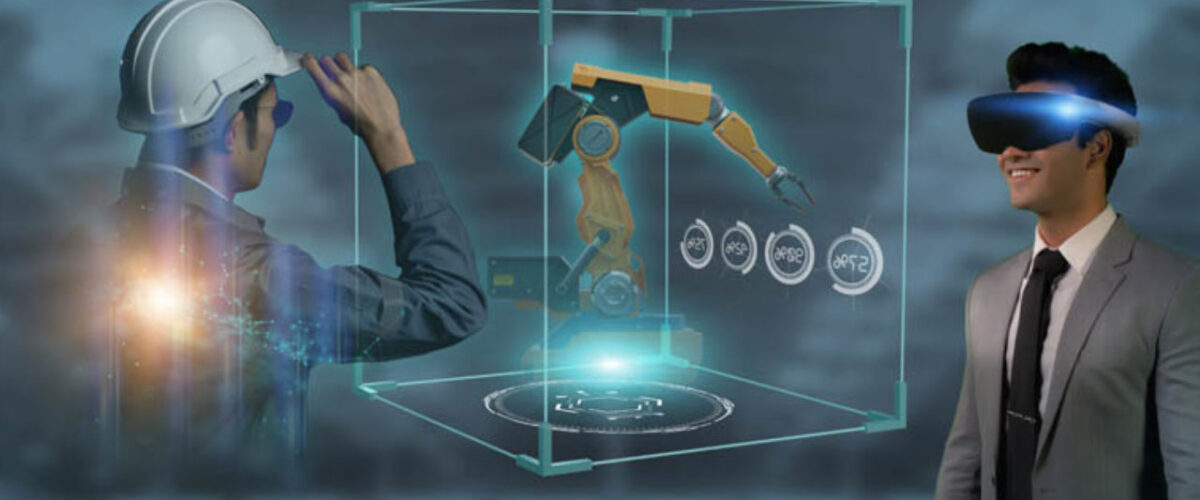
Generative Artificial Intelligence (AI) represents a revolutionary approach to AI systems that can generate new and original content, such as images, music, text, and even videos. Unlike traditional AI models that rely on predefined rules and patterns, generative AI models have the ability to learn from existing data and create new content that resembles the training examples.
Generative AI models leverage deep learning techniques, such as deep neural networks and generative adversarial networks (GANs), to understand and replicate patterns in the data they are trained on. They can then generate novel outputs by extrapolating from the learned patterns or by combining and transforming existing data in innovative ways.
The creative potential of generative AI is vast, and it has found applications in various domains. Generative AI models can produce realistic images that resemble photographs, generate music compositions, write stories or poetry, and even design new virtual objects or characters. By mimicking human creativity and ingenuity, generative AI is pushing the boundaries of what machines can achieve.
Generative AI has also contributed to the emergence of new forms of artistic expression known as “generative art.” Artists and creative professionals are utilizing generative AI tools and techniques to explore new aesthetic possibilities and create artwork that challenges traditional artistic conventions. Generative AI has become a valuable tool for artists to collaborate with machines in the creative process, allowing for the creation of unique and thought-provoking pieces.
Moreover, generative AI has practical applications beyond the creative realm. It can aid in data augmentation and synthesis, facilitating the creation of realistic and diverse datasets for training machine learning models. Generative AI models are also used in various industries, including gaming, fashion, advertising, and design, to generate personalized recommendations, simulate virtual environments, and assist in product development.
As generative AI continues to advance, researchers and developers are exploring new techniques, architectures, and training methodologies to improve the quality, diversity, and control of generated outputs. Ethical considerations, such as bias mitigation and responsible use of generative AI, are also important topics of discussion as these models gain more prominence.
In summary, generative AI represents a transformative approach to AI that goes beyond rule-based systems to enable machines to generate new and original content. Its creative potential and practical applications make it an exciting field of research and development, with implications across various industries and artistic domains.
Role of Generative AI in Augmented Reality and Mixed Reality Experiences
Generative AI plays a crucial role in shaping augmented reality (AR) and mixed reality (MR) experiences, enhancing their realism, interactivity, and immersion. By leveraging generative AI techniques, AR and MR applications can generate virtual content, modify real-world scenes, and provide interactive elements that seamlessly blend with the physical environment. Here are some key roles of generative AI in AR and MR experiences:
- Virtual Object Generation: Generative AI enables the creation of virtual objects and entities that can be placed and interacted with in the real world. By training on existing datasets or models, generative AI can synthesize virtual characters, creatures, or objects with realistic appearances, behaviors, and movements. This enhances the visual fidelity and engagement of AR and MR experiences.
- Real-time Environment Mapping: Generative AI techniques, such as Simultaneous Localization and Mapping (SLAM), enable the real-time mapping and understanding of the physical environment. By combining sensor data with generative models, AR and MR applications can accurately track the user’s position and orientation relative to the surroundings, facilitating the alignment and integration of virtual content with the real world.
- Object Recognition and Tracking: Generative AI models can be trained to recognize and track specific objects or features in the environment. This enables AR and MR applications to overlay virtual information or visual enhancements onto recognized objects, enhancing their appearance or providing additional context-related information.
- Realistic Visual Effects: Generative AI can enhance the visual effects and realism of AR and MR experiences. By leveraging techniques like image synthesis, super-resolution, or style transfer, generative AI models can improve the visual quality of captured or virtual content, making it more immersive and visually appealing.
- Dynamic Content Adaptation: Generative AI models can adapt virtual content dynamically based on the real-time analysis of the environment and user interactions. For example, virtual objects can react to the user’s movements, respond to changes in lighting conditions, or interact with real-world objects, creating a more interactive and responsive AR or MR experience.
- Scene Reconstruction and Simulation: Generative AI can reconstruct and simulate virtual scenes based on real-world environments. By analyzing sensor data and using generative models, AR and MR applications can generate realistic virtual representations of the physical surroundings, allowing users to explore and interact with augmented elements seamlessly.
- User Personalization and Customization: Generative AI enables personalized AR and MR experiences by leveraging user data and preferences. By analyzing user behavior, preferences, or biometric data, generative AI models can adapt and customize the content or interactions within AR and MR applications, creating tailored experiences for individual users.
The integration of generative AI techniques into AR and MR experiences enhances their capabilities, making them more immersive, interactive, and contextually aware. As generative AI continues to advance, we can expect further improvements in the realism, responsiveness, and personalized nature of AR and MR applications, unlocking new possibilities for entertainment, education, training, design, and various other domains.
Conclusion
In conclusion, generative AI plays a significant and transformative role in shaping augmented reality (AR) and mixed reality (MR) experiences. By leveraging advanced generative techniques, AR and MR applications can generate virtual content, seamlessly integrate it into the real world, and enhance the overall user experience.
Generative AI enables the creation of virtual objects and entities that appear realistic and behave authentically, enhancing the visual fidelity and engagement of AR and MR experiences. It also facilitates real-time environment mapping and object recognition, allowing virtual content to interact with and respond to the physical surroundings, creating a more immersive and interactive experience.
The use of generative AI models enhances the visual effects and realism of AR and MR by improving the quality of captured or virtual content. It enables dynamic content adaptation, where virtual objects can react and respond to user movements, lighting conditions, or real-world objects, providing a more responsive and contextually aware experience.
Generative AI also contributes to scene reconstruction and simulation, generating realistic virtual representations of the physical environment based on sensor data. This allows users to explore and interact with augmented elements seamlessly, blurring the lines between the real and virtual worlds.
Furthermore, generative AI enables user personalization and customization by leveraging user data and preferences. AR and MR applications can adapt and customize the content or interactions based on individual user behavior and preferences, creating tailored experiences that cater to specific user needs.
As generative AI continues to advance, we can anticipate further improvements in the capabilities of AR and MR experiences. The integration of generative AI techniques will lead to more immersive, interactive, and contextually aware applications, offering enhanced entertainment, education, training, design, and other possibilities across various domains.
Overall, the role of generative AI in AR and MR experiences is pivotal, transforming these technologies into powerful tools for delivering captivating, interactive, and personalized augmented experiences that bridge the gap between the physical and virtual worlds.
Post a Comment