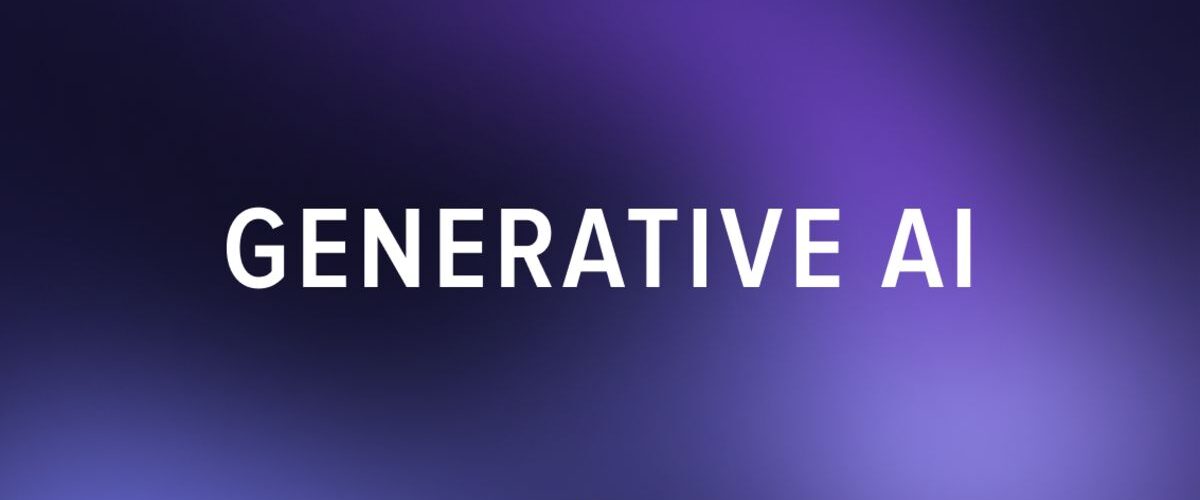
Generative AI, with its ability to create and synthesize new content, has immense potential in various domains, including art, entertainment, design, and more. However, as with any powerful technology, there are important ethical considerations that need to be addressed. The capabilities of generative AI raise concerns related to privacy, fairness, ownership, accountability, and societal impact. It is crucial to examine these ethical considerations and navigate the development and deployment of generative AI systems responsibly.
Privacy is a significant concern when it comes to generative AI. The technology has the potential to generate highly realistic and convincing content, which can be used to create deepfakes or manipulate personal data. Safeguarding individual privacy and ensuring the responsible use of generative AI systems is essential to protect individuals from potential harm or misuse.
Fairness and bias are also critical considerations in generative AI. The models used for training can inadvertently perpetuate biases present in the training data, leading to unfair or discriminatory outcomes in generated content. It is essential to ensure that the training data is diverse, representative, and free from biases, and to develop mechanisms that mitigate and address any biases that may emerge during the training process.
Ownership and intellectual property rights are additional ethical concerns in generative AI. With the ability to generate content that resembles existing works, questions arise regarding who owns the generated content and how it can be used. Establishing clear guidelines and frameworks for ownership, attribution, and copyright in the context of generative AI is crucial to protect the rights of creators, prevent plagiarism, and promote fair use of generated content.
Accountability and transparency are important aspects of ethical generative AI. It is essential to understand the decision-making processes of generative AI systems and ensure that they can be audited and explained. Developers should strive to make the operation of generative AI systems transparent, enabling users and stakeholders to understand how the system generates content and to detect any potential biases or flaws.
Lastly, the societal impact of generative AI must be carefully considered. While generative AI has the potential to enhance creativity, entertainment, and innovation, it can also have unintended consequences. It is essential to proactively assess and mitigate potential societal risks and negative impacts that may arise from the use of generative AI. Collaborative efforts between researchers, developers, policymakers, and other stakeholders are necessary to ensure that generative AI technologies are developed and deployed in a manner that benefits society as a whole.
Addressing these ethical considerations requires a multidisciplinary approach, involving experts in AI, ethics, law, policy, and various other fields. It is essential to strike a balance between the potential benefits of generative AI and the ethical responsibilities associated with its development and use. By proactively addressing these ethical considerations, we can foster the responsible development and deployment of generative AI systems that align with societal values, protect individual rights, and contribute positively to the well-being of individuals and communities.
Bias and Fairness of Generative AI
Bias and fairness are significant ethical considerations in the development and deployment of generative AI systems. Generative AI, with its ability to create new content, has the potential to perpetuate and amplify biases present in the training data, leading to unfair or discriminatory outcomes in the generated content. It is crucial to address bias and ensure fairness to mitigate potential harm and promote equitable and inclusive use of generative AI.
Here are some key points to consider regarding bias and fairness in generative AI:
- Bias in Training Data: Generative AI models learn from large datasets, which can inadvertently reflect biases present in the data. If the training data is unrepresentative or biased, the generated content may also exhibit similar biases. For example, if the training data is predominantly of a certain demographic group, the generated content may disproportionately represent that group, leading to underrepresentation or misrepresentation of other groups.
- Amplification of Stereotypes: Generative AI models can unintentionally amplify stereotypes and biases that exist in society. For instance, if a model is trained on biased text data, it may generate biased or offensive language. Similarly, if a model is trained on biased image data, it may generate images that reinforce stereotypes related to gender, race, or other attributes.
- Fairness in Generated Outputs: Fairness considerations should be applied to the outputs of generative AI systems. This includes ensuring that the generated content does not favor or discriminate against specific individuals or groups based on protected attributes such as race, gender, age, or religion. It is crucial to prevent the generation of content that promotes hate speech, discrimination, or harm.
- Diverse and Representative Training Data: To address bias and promote fairness, it is important to use diverse and representative training data. This means including data from a wide range of sources and perspectives, ensuring proper representation of different demographic groups, cultures, and viewpoints. By incorporating diverse training data, generative AI models can learn to generate content that is more inclusive and less biased.
- Bias Mitigation Techniques: Researchers and developers are actively exploring techniques to mitigate bias in generative AI. These techniques include debiasing algorithms, data augmentation, algorithmic fairness frameworks, and adversarial training methods. By incorporating such techniques, it is possible to reduce the influence of biases in the training data and promote fairer outcomes in the generated content.
- User Control and Customization: Providing users with control and customization options can enhance fairness in generative AI systems. Allowing users to influence the generated content based on their preferences, values, and requirements can empower them to mitigate biases and ensure content that aligns with their desired outcomes.
- Ethical Guidelines and Standards: The development and deployment of generative AI should adhere to ethical guidelines and standards that explicitly address bias and fairness. These guidelines should emphasize the importance of fairness, inclusivity, and non-discrimination, providing clear instructions and best practices for developers and users of generative AI systems.
Addressing bias and ensuring fairness in generative AI requires a proactive and multidimensional approach. It involves careful consideration of training data, algorithmic design, user interfaces, and evaluation metrics. Collaboration among researchers, developers, policymakers, and stakeholders from diverse backgrounds is crucial to effectively identify and mitigate biases, promote fairness, and create generative AI systems that uphold ethical standards and societal values.
Ethical Considerations in Generative AI
Generative AI, with its ability to create new content and simulate human-like behavior, brings forth a range of ethical considerations that need to be carefully addressed. These considerations span various aspects, including privacy, intellectual property, bias and fairness, accountability, transparency, and potential societal impact. By understanding and addressing these ethical considerations, we can ensure the responsible development and use of generative AI systems.
- Privacy: Generative AI has the potential to generate realistic content that can infringe upon individuals’ privacy. Deepfakes, for example, raise concerns about unauthorized manipulation and misuse of personal data. It is important to establish safeguards and guidelines to protect individuals’ privacy rights and prevent the malicious use of generative AI technology.
- Intellectual Property: Generative AI systems have the capability to create content that resembles existing works, raising questions about copyright infringement and ownership. Clear guidelines and frameworks are needed to determine the ownership and attribution of generated content, as well as to address issues related to plagiarism and fair use.
- Bias and Fairness: Bias can inadvertently be present in the training data used to train generative AI models, which can lead to biased outputs. Ensuring fairness and mitigating bias is crucial to prevent the generation of discriminatory or offensive content. Robust techniques, diverse training data, and thorough evaluation processes are necessary to address bias and promote fair outcomes.
- Accountability and Transparency: Generative AI systems often involve complex algorithms and decision-making processes. It is important to ensure that these systems can be audited, understood, and held accountable for their outputs and behaviors. Transparency in how generative AI systems operate, including their training processes, data sources, and algorithms, can foster trust and enable responsible use.
- Social and Ethical Impact: Generative AI can have wide-ranging societal impacts, both positive and negative. It is essential to carefully consider the potential consequences and implications of deploying generative AI systems. This includes assessing the potential for job displacement, economic implications, cultural impact, and effects on human creativity and expression. Ethical frameworks and impact assessments can help navigate these complex considerations.
- Human Consent and Control: In certain applications of generative AI, such as deepfakes, consent and control become critical. Respect for individual autonomy and consent should be upheld, ensuring that individuals have control over the use of their personal data and how it is represented or manipulated by generative AI systems.
- Dual-Use and Ethical Guidelines: Generative AI technology can have both positive and negative applications. It is important to establish ethical guidelines and frameworks that address the potential dual-use nature of generative AI and promote responsible development and deployment. These guidelines can help guide developers, users, and policymakers in making ethical decisions and ensuring the technology is used in a manner that aligns with societal values.
Conclusion
In conclusion, ethical considerations in generative AI, specifically addressing bias and fairness, are crucial for responsible development and deployment. The potential for biased outputs and unfair outcomes poses significant risks, impacting privacy, intellectual property, and societal well-being. By proactively addressing these concerns, we can ensure that generative AI systems contribute positively to society while minimizing harm.
To address bias and promote fairness, it is essential to focus on diverse and representative training data, employing techniques such as debiasing algorithms and adversarial training. Clear guidelines and frameworks for ownership, attribution, and copyright are necessary to navigate intellectual property issues. Transparency and accountability in the operation of generative AI systems enhance user trust and allow for meaningful audits and explanations.
The involvement of diverse stakeholders, including researchers, developers, policymakers, ethicists, and impacted communities, is crucial for establishing ethical guidelines and standards. Collaborative efforts can lead to the development of robust evaluation metrics and frameworks that mitigate biases and ensure fairness in the generated content.
Moreover, ethical considerations extend beyond technical aspects. They involve informed consent, user control, and promoting human values and rights. Striking a balance between the potential benefits of generative AI and the ethical responsibilities associated with its use is paramount.
By addressing bias and fairness in generative AI, we can mitigate the risks of discriminatory or harmful outputs and foster an inclusive and equitable AI ecosystem. Ethical guidelines, responsible practices, and ongoing research are necessary to ensure that generative AI systems are developed and used in a manner that respects privacy, promotes fairness, and aligns with societal values, ultimately maximizing the positive impact of this transformative technology.
Post a Comment