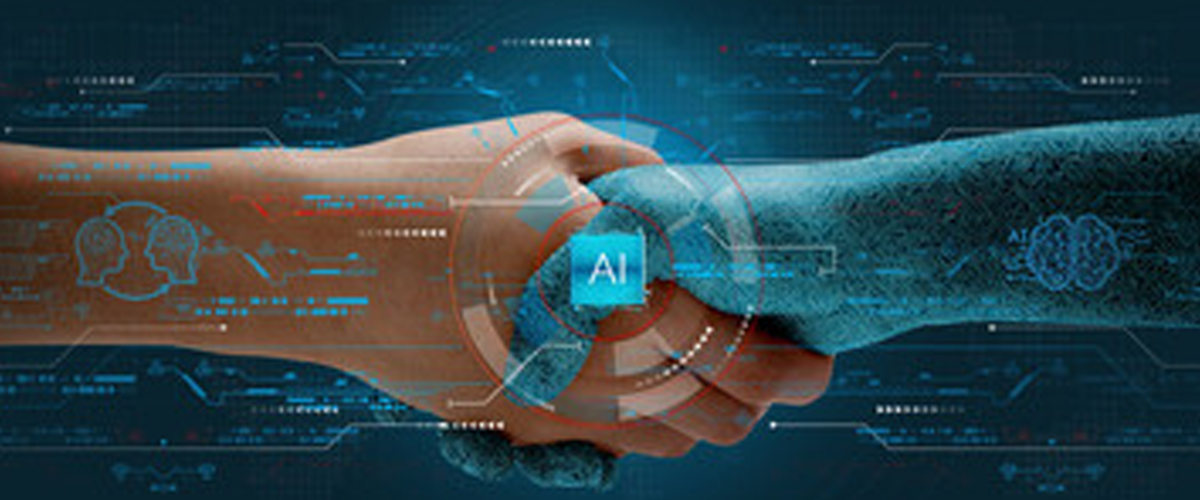
A form of artificial intelligence known as “generative AI” focuses on producing new content or data, such as text, photos, music, or videos, as opposed to only recognizing or processing already-existing data. Machines can learn from a dataset, spot patterns and relationships, and then utilize that knowledge to create new data that closely resembles the properties of the original dataset thanks to generative AI algorithms.
Reinforcement learning, unsupervised learning, and supervised learning are just a few of the methods that generative AI models might utilize to generate new material that is both authentic and unique. In addition to text and speech generation, generative AI is frequently used to create art, music, and video.
By enabling the generation of distinctive and individualized content for customers, generative AI has the potential to completely transform a variety of industries, including entertainment, advertising, and e-commerce. The need to verify that the generated content is morally upright, objective, and does not violate copyright or other intellectual property rights, for example, is one of the new difficulties it brings.
How Do Generative AI Functions?
Generative AI creates new content that is comparable to the original data by using sophisticated algorithms and machine learning approaches to extract patterns and relationships from a dataset. Here is a general explanation of how generative AI operates:
- Training the model: In generative AI, the initial stage is to train a deep neural network on a sizable dataset (such as photos, text, music, etc.). Large amounts of data are needed for this training procedure, often labeled data (supervised learning) like photos with pertinent tags or text with sentiment ratings. To produce a probabilistic understanding of its features, the model learns the correlations and patterns in this data.
- Once the model has been trained, it can begin producing fresh content. It accomplishes this by first mapping a random input (sometimes referred to as a latent vector) to a new output. A generative AI system, for instance, may utilize a face image to create a new face or some text to create new text.
- Results that are improved: As with any learning system, the model’s output might not be ideal or even desirable for the task at hand. As a result, scientists and programmers can keep modifying the model’s hyperparameters and training it on more datasets to achieve the best results.
Overall, generative AI combines large datasets, complex algorithms, and machine learning techniques to create something entirely new, providing exciting opportunities to businesses and researchers looking to explore creative and innovative ways of producing new content.
What are the use cases for Generative AI?
Generative AI is incredibly versatile, and its potential applications continue to expand as technology moves forward. Here are some of the most exciting use cases for generative AI:
- Art and design: Generative AI can produce new, beautiful, and original images, music, videos, and other types of media. For instance, AI-generated artwork has appeared in commercials, music videos, and even exhibitions.
- Gaming and virtual worlds: Generative AI can be used to build dynamic, intricate settings where players can interact with people, objects, and other things. This entails generating fresh game concepts and maps for video games as well as placing AI-generated residents in those locations.
- Personalization: Based on a consumer’s preferences or past behavior, generative AI can create content that is tailored to that specific consumer. This involves making recommendations for new goods or services, creating tailored marketing campaigns, and creating dynamic content for websites and mobile applications.
- Text and Language: Generative AI can be used to produce original writing, speech, translations, summaries, and even dialogue for virtual assistants or chatbots.
- Security: By learning from past attacks and creating new situations, generative AI may be used to simulate possible cyberattacks and discover potential security issues.
- Healthcare & Medicine: Using extensive datasets from medical records, generative AI can develop novel medication therapies, personalized medicines, and predictive health models that can precisely spot patterns and trends in patient data.
Benefits of Generative AI
Generative AI offers several benefits, some of which include:
- Increased Productivity: The automation of many jobs using generative AI can boost productivity, efficiency, and eliminate human errors.
- Personalization: Generative AI can produce tailored content for specific users based on their likes, dislikes, and previous interactions, which can help to boost engagement and client happiness.
- New Product Development: Generative AI can aid with the creation of new and original designs or prototypes for new items, which can save firms time and money.
- Finding Hidden Insights: Generative AI can assist in finding links and patterns in vast, complicated datasets that may not be immediately apparent to human analysts, which can lead to new knowledge.
- Enhanced Creativity: Generative AI can provide fresh, original content that can assist boost creativity across a variety of businesses, including the creative and advertising industries.
- Predictive Analysis: Generative AI algorithms have the ability to analyze enormous amounts of historical data in order to forecast future trends and patterns, assisting organizations in making strategic decisions about their operations.
How Generative AI is Governed?
Experts in the area, decision-makers, and regulatory authorities are currently debating and discussing the complicated topic of generative AI governance. There is a need for ethical and responsible development, implementation, and use of Generative AI given its tremendous capabilities and potential social repercussions.
A wide range of stakeholders, including AI researchers, Generative AI-using organizations, legislators, and the general public, must be included in order to properly control Generative AI. Generative AI must be governed in a transparent and cooperative manner to guarantee that it is created and applied in a responsible and safe manner.
The most prominent current efforts to govern Generative AI are:
- Industry Self-Regulation: Organizations inside the industry, such as the Partnership on AI or IEEE, work to establish standards, moral concerns, and best practices that should be adhered to when developing and using generative artificial intelligence.
- Government Regulation: Laws, rules, and policies to control the use of generative artificial intelligence are being developed by governments all over the world.
- Education and Public Awareness: The public needs to be made aware of the possible benefits and risks of generative AI technology. People are becoming more and more interested in learning about ethical issues and potential advantages so they may assess their options and make wise judgements.
- International Cooperation: To ensure that technology may be utilized safely, ethically, and responsibly, international cooperation is crucial throughout the development and deployment of generative AI.
Know About the Limitations of Generative AI
Despite its impressive capabilities, Generative AI also has a few limitations. Some key limitations include:
- Data Availability and Quality: To train efficiently, generative AI models need a lot of high-quality data. The accuracy and quality of created content may suffer in the absence of sufficient data.
- Concerns about bias and ethics: Generative AI may provide biased or discriminating results since it may pick up prejudices from the data sets it is trained on. To achieve equitable results, it is crucial that concerns relating to bias, justice, and ethics be taken into account and addressed when developing generative AI.
- Control over Output: Once a Generative AI model has been trained and put into operation, it can produce material independently of the people who created it, which opens the possibility of data misuse or misrepresentation.
- Powerful processing gear is needed for Generative AI models because of their complexity, which can be expensive and energy-intensive.
- Lack of Interpretability: Generative AI models can be challenging to grasp, making it challenging to pinpoint problems when something goes wrong or comprehend how decisions are being made.
Conclusion
In conclusion, the topic of generative AI is quickly developing and has the potential to revolutionize a variety of sectors and everyday life. It has created new avenues for the creation of art, literature, music, and even artificially intelligent virtual people. The potential ethical issues and repercussions must be thoroughly explored and addressed, though, as with any powerful technology. Researchers, governments, and society as a whole must collaborate as the field develops to make sure that generative AI is applied in ways that benefit humanity while minimizing any unfavorable effects.
Post a Comment